Running your R script in Docker
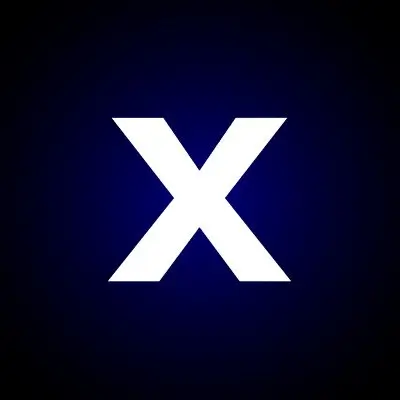

Since its release in 2014, Docker has become an essential tool for deploying applications. At statworx, R is part of our daily toolset. Clearly, many of us were thrilled to learn about RStudio’s Rocker Project, which makes containerizing R code easier than ever.
Containerization is useful in a lot of different situations. To me, it is very helpful when I’m deploying R code in a cloud computing environment, where the coded workflow needs to be run on a regular schedule. Docker is a perfect fit for this task for two reasons: On the one hand, you can simply schedule a container to be started at your desired interval. On the other hand, you always know what behavior and what output to expect, because of the static nature of containers. So if you’re tasked with deploying a machine-learning model that should regularly make predictions, consider doing so with the help of Docker. This blog entry will guide you through the entire process of getting your R script to run in a Docker container one step at a time. For the sake of simplicity, we’ll be working with a local dataset.
I’d like to start off with emphasizing that this blog entry is not a general Docker tutorial. If you don’t really know what images and containers are, I recommend that you take a look at the Docker Curriculum first. If you’re interested in running an RStudio session within a Docker container, then I suggest you pay a visit to the OpenSciLabs Docker Tutorial instead. This blog specifically focuses on containerizing an R script to eventually execute it automatically each time the container is started, without any user interaction – thus eliminating the need for the RStudio IDE. The syntax used in the Dockerfile and the command line will only be treated briefly here, so it’s best to get familiar with the basics of Docker before reading any further.
What we’ll need
For the entire procedure we’ll be needing the following:
- An R script which we’ll build into an image
- A base image on top of which we’ll build our new image
- A Dockerfile which we’ll use to build our new image
You can clone all following files and the folder structure I used from the statworx GitHub Repository.

The R script
We’re working with a very simple R script that imports a dataframe, manipulates it, creates a plot based on the manipulated data and, in the end, exports both the plot and the data it is based on. The dataframe used for this example is the US 500 Records dataset provided by Brian Dunning. If you’d like to work along, I’d recommend you to copy this dataset into the 01_data folder.
library(readr)
library(dplyr)
library(ggplot2)
library(forcats)
# import dataframe
df <- read_csv("01_data/us-500.csv")
# manipulate data
plot_data <- df %>%
group_by(state) %>%
count()
# save manipulated data to output folder
write_csv(plot_data, "03_output/plot_data.csv")
# create plot based on manipulated data
plot <- plot_data %>%
ggplot()+
geom_col(aes(fct_reorder(state, n),
n,
fill = n))+
coord_flip()+
labs(
title = "Number of people by state",
subtitle = "From US-500 dataset",
x = "State",
y = "Number of people"
)+
theme_bw()
# save plot to output folder
ggsave("03_output/myplot.png", width = 10, height = 8, dpi = 100)
This creates a simple bar plot based on our dataset:

We use this script not only to run R code inside a Docker container, but we also want to run it on data from outside our container and afterward save our results.
The base image
The DockerHub page of the Rocker project lists all available Rocker repositories. Seeing as we’re using Tidyverse-packages in our script the rocker/tidyverse
image should be an obvious choice. The problem with this repository is that it also includes RStudio, which is not something we want for this specific project. This means that we’ll have to work with the r-base
repository instead and build our own Tidyverse-enabled image. We can pull the rocker/r-base
image from DockerHub by executing the following command in the terminal:
docker pull rocker/r-base
This will pull the Base-R image from the Rocker DockerHub repository. We can run a container based on this image by typing the following into the terminal:
docker run -it --rm rocker/r-base
Congratulations! You are now running R inside a Docker container! The terminal was turned into an R console, which we can now interact with thanks to the -it
argument. The —-rm
argument makes sure the container is automatically removed once we stop it. You’re free to experiment with your containerized R session, which you can exit by executing the q()
function from the R console. You could, for example, start installing the packages you need for your workflow with install.packages()
, but that’s usually a tedious and time-consuming task. It is better to already build your desired packages into the image, so you don’t have to bother with manually installing the packages you need every time you start a container. For that, we need a Dockerfile.
The Dockerfile
With a Dockerfile, we tell Docker how to build our new image. A Dockerfile is a text file that must be called “Dockerfile.txt” and by default is assumed to be located in the build-context root directory (which in our case would be the “R-Script in Docker” folder). First, we have to define the image on top of which we’d like to build ours. Depending on how we’d like our image to be set up, we give it a list of instructions so that running containers will be as smooth and efficient as possible. In this case, I’d like to base our new image on the previously discussed rocker/r-base
image. Next, we replicate the local folder structure, so we can specify the directories we want in the Dockerfile. After that we copy the files which we want our image to have access to into said directories – this is how you get your R script into the Docker image. Furthermore, this allows us to prevent having to manually install packages after starting a container, as we can prepare a second R script that takes care of the package installation. Simply copying the R script is not enough, we also need to tell Docker to automatically run it when building the image. And that’s our first Dockerfile!
# Base image https://hub.docker.com/u/rocker/
FROM rocker/r-base:latest
## create directories
RUN mkdir -p /01_data
RUN mkdir -p /02_code
RUN mkdir -p /03_output
## copy files
COPY /02_code/install_packages.R /02_code/install_packages.R
COPY /02_code/myScript.R /02_code/myScript.R
## install R-packages
RUN Rscript /02_code/install_packages.R
Don’t forget preparing and saving your appropriate install_packages.R
script, where you specify which R packages you need to be pre-installed in your image. In our case the file would look like this:
install.packages("readr")
install.packages("dplyr")
install.packages("ggplot2")
install.packages("forcats")
Building and running the image
Now we have assembled all necessary parts for our new Docker image. Use the terminal to navigate to the folder where your Dockerfile is located and build the image with
docker build -t myname/myimage .
The process will take a while due to the package installation. Once it’s finished we can test our new image by starting a container with
docker run -it --rm -v ~/"R-Script in Docker"/01_data:/01_data -v ~/"R-Script in Docker"/03_output:/03_output myname/myimage
Using the -v
arguments signales Docker which local folders to map to the created folders inside the container. This is important because we want to both get our dataframe inside the container and save our output from the workflow locally so it isn’t lost once the container is stopped.
This container can now interact with our dataframe in the 01_data folder and has a copy of our workflow-script inside its own 02_code folder. Telling R to source("02_code/myScript.R")
will run the script and save the output into the 03_output folder, from where it will also be copied to our local 03_output folder.

Improving on what we have
Now that we have tested and confirmed that our R script runs as expected when containerized, there’s only a few things missing.
- We don’t want to manually have to source the script from inside the container, but have it run automatically whenever the container is started.
We can achieve this very easily by simply adding the following command to the end of our Dockerfile:
## run the script
CMD Rscript /02_code/myScript.R
This points towards the location of our script within the folder structure of our container, marks it as R code and then tells it to run whenever the container is started. Making changes to our Dockerfile, of course, means that we have to rebuild our image and that in turn means that we have to start the slow process of pre-installing our packages all over again. This is tedious, especially if chances are that there will be further revisions of any of the components of our image down the road. That’s why I suggest we
- Create an intermediary Docker image where we install all important packages and dependencies so that we can then build our final, desired image on top.
This way we can quickly rebuild our image within seconds, which allows us to freely experiment with our code without having to sit through Docker installing packages over and over again.
Building an intermediary image
The Dockerfile for our intermediary image looks very similar to our previous example. Because I decided to modify my install_packages()
script to include the entire tidyverse for future use, I also needed to install a few debian packages the tidyverse depends upon. Not all of these are 100% necessary, but all of them should be useful in one way or another.
# Base image https://hub.docker.com/u/rocker/
FROM rocker/r-base:latest
# system libraries of general use
## install debian packages
RUN apt-get update -qq && apt-get -y --no-install-recommends install \
libxml2-dev \
libcairo2-dev \
libsqlite3-dev \
libmariadbd-dev \
libpq-dev \
libssh2-1-dev \
unixodbc-dev \
libcurl4-openssl-dev \
libssl-dev
## update system libraries
RUN apt-get update && \
apt-get upgrade -y && \
apt-get clean
## create directories
RUN mkdir -p /02_code
## copy files
COPY /02_code/install_packages.R /02_code/install_packages.R
## install R-packages
RUN Rscript /02_code/install_packages.R
I build the image by navigating to the folder where my Dockerfile sits and executing the Docker build command again:
docker build -t oliverstatworx/base-r-tidyverse .
I have also pushed this image to my DockerHub so if you ever need a base-R image with the tidyverse pre-installed you can simply build it ontop of my image without having to go through the hassle of building it yourself.
Now that the intermediary image has been built we can change our original Dockerfile to build on top of it instead of rocker/r-base
and remove the package-installation because our intermediary image already takes care of that. We also add the last line that automatically starts running our script whenever the container is started. Our final Dockerfile should look something like this:
# Base image https://hub.docker.com/u/oliverstatworx/
FROM oliverstatworx/base-r-tidyverse:latest
## create directories
RUN mkdir -p /01_data
RUN mkdir -p /02_code
RUN mkdir -p /03_output
## copy files
COPY /02_code/myScript.R /02_code/myScript.R
## run the script
CMD Rscript /02_code/myScript.R
The final touches
Since we built our image on top of an intermediary image with all our needed packages, we can now easily modify parts of our final image to our liking. I like making my R script less verbose by suppressing warnings and messages that are not of interest anymore (since I already tested the image and know that everything works as expected) and adding messages that tell the user which part of the script is currently being executed by the running container.
suppressPackageStartupMessages(library(readr))
suppressPackageStartupMessages(library(dplyr))
suppressPackageStartupMessages(library(ggplot2))
suppressPackageStartupMessages(library(forcats))
options(scipen = 999,
readr.num_columns = 0)
print("Starting Workflow")
# import dataframe
print("Importing Dataframe")
df <- read_csv("01_data/us-500.csv")
# manipulate data
print("Manipulating Data")
plot_data <- df %>%
group_by(state) %>%
count()
# save manipulated data to output folder
print("Writing manipulated Data to .csv")
write_csv(plot_data, "03_output/plot_data.csv")
# create plot based on manipulated data
print("Creating Plot")
plot <- plot_data %>%
ggplot()+
geom_col(aes(fct_reorder(state, n),
n,
fill = n))+
coord_flip()+
labs(
title = "Number of people by state",
subtitle = "From US-500 dataset",
x = "State",
y = "Number of people"
)+
theme_bw()
# save plot to output folder
print("Saving Plot")
ggsave("03_output/myplot.png", width = 10, height = 8, dpi = 100)
print("Worflow Finished")
After navigating to the folder where our Dockerfile is located we rebuild our image once more with: docker build -t myname/myimage .
Once again we start a container based on our image and map the 01_data and 03_output folders to our local directories. This way we can import our data and save our created output locally:
docker run -it --rm -v ~/"R-Script in Docker"/01_data:/01_data -v ~/"R-Script in Docker"/03_output:/03_output myname/myimage
Congratulations, you now have a clean Docker image that not only automatically runs your R script whenever a container is started, but also tells you exactly which part of the code it is executing via console messages. Happy docking!