Paradigm Shift in NLP: 5 Approaches to Write Better Prompts
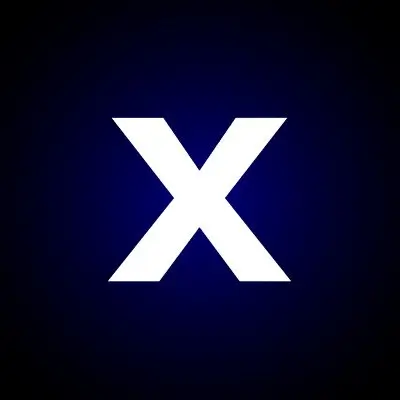

At statworx, we deal intensively with how to get the best possible results from large language models (LLMs). In this blog post, I present five approaches that have proven successful both in research and in our own work with LLMs. While this text is limited to the manual design of prompts for text generation, image generation and automated prompt search will be the topic of future posts.
Mega models herald new paradigm
The arrival of the revolutionary language model GPT-3 was not only a turning point for the research field of language modeling (NLP) but has incidentally heralded a paradigm shift in AI development: prompt learning. Prior to GPT-3, the standard was fine-tuning of medium-sized language models such as BERT, which, thanks to re-training with new data, would adapt the pre-trained model to the desired use case. Such fine-tuning requires exemplary data for the desired application, as well as the computational capabilities to at least partially re-train the model.
The new large language models such as OpenAI’s GPT-3 and BigScience’s BLOOM, on the other hand, have already been trained by their development teams with such enormous amounts of resources that these models have achieved a new level of independence in their intended use: These LLMs no longer require elaborate fine-tuning to learn their specific purpose, but already produce impressive results using targeted instruction (“prompt”) in natural language.
So, we are in the midst of a revolution in AI development: Thanks to prompt learning, interaction with models no longer takes place via code, but in natural language. This is a giant step forward for the democratization of language modeling. Generating text or, most recently, even creating images requires no more than rudimentary language skills. However, this does not mean that compelling or impressive results are accessible to all. High quality outputs require high quality inputs. For us users, this means that engineering efforts in NLP are no longer focused on model architecture or training data, but on the design of the instructions that models receive in natural language. Welcome to the age of prompt engineering.

Figure 1: From prompt to prediction with a large language model.
Prompts are more than just snippets of text
Templates facilitate the handling of prompts
Since LLMs have not been trained on a specific use case, it is up to the prompt design to provide the model with the exact task. So-called “prompt templates” are used for this purpose. A template defines the structure of the input that is passed on to the model. Thus, the template takes over the function of fine-tuning and determines the expected output of the model for a specific use case. Using sentiment analysis as an example, a simple prompt template might look like this:
The expressed sentiment in text [X] is: [Z]
The model thus searches for a token z, that, based on the trained parameters and the text in location [X], maximizes the probability of the masked token in location [Z]. The template thus specifies the desired context of the problem to be solved and defines the relationship between the input at position [X] and the output to be predicted at position [Z]. The modular structure of templates enables the systematic processing of a large number of texts for the desired use case.
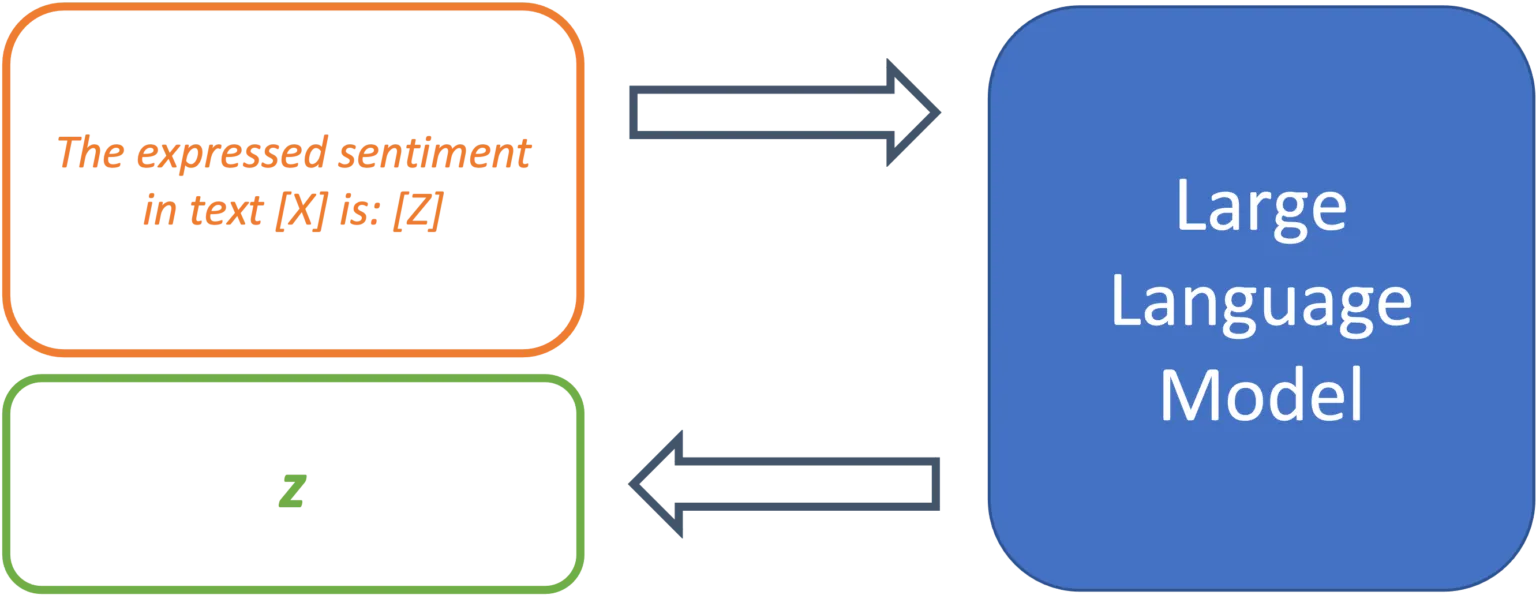
Figure 2: Prompt templates define the structure of a prompt.
Prompts do not necessarily need examples
The template presented is an example of a so-called “0-Shot” prompt, since there is only an instruction, without any demonstration with examples in the template. Originally, LLMs were called “Few-Shot Learners” by the developers of GPT-3, i.e., models whose performance can be maximized with a selection of solved examples of the problem (Brown et al., 2020). However, a follow-up study showed that with strategic prompt design, 0-shot prompts, without a large number of examples, can achieve comparable performance (Reynolds & McDonell, 2021). Thus, since different approaches are also used in research, the next section presents 5 strategies for effective prompt template design.
5 Strategies for Effective Prompt Design
Task demonstration
In the conventional few-shot setting, the problem to be solved is narrowed down by providing several examples. The solved example cases are supposed to take a similar function as the additional training samples during the fine-tuning process and thus define the specific use case of the model. Text translation is a common example for this strategy, which can be represented with the following prompt template:
French: „Il pleut à Paris“
English: „It’s raining in Paris“
French: „Copenhague est la capitale du Danemark“
English: „Copenhagen is the capital of Denmark“
[…]
French: [X]
English: [Z]
While the solved examples are good for defining the problem setting, they can also cause problems. “Semantic contamination” refers to the phenomenon of the LLM interpreting the content of the translated sentences as relevant to the prediction. Examples in the semantic context of the task produce better results – and those out of context can lead to the prediction Z being “contaminated” in terms of its content (Reynolds & McDonell, 2021). Using the above template for translating complex facts, the model might well interpret the input sentence as a statement about a major European city in ambiguous cases.
Task Specification
Recent research shows that with good prompt design, even the 0-shot approach can yield competitive results. For example, it has been demonstrated that LLMs do not require pre-solved examples at all, as long as the problem is defined as precisely as possible in the prompt (Reynolds & McDonell, 2021). This specification can take different forms, but it is always based on the same idea: to describe as precisely as possible what is to be solved, but without demonstrating how.
A simple example of the translation case would be the following prompt:
Translate from French to English [X]: [Z]
This may already work, but the researchers recommend making the prompt as descriptive as possible and explicitly mentioning translation quality:
A French sentence is provided: [X]. The masterful French translator flawlessly translates the sentence to English: [Z]
This helps the model locate the desired problem solution in the space of the learned tasks.
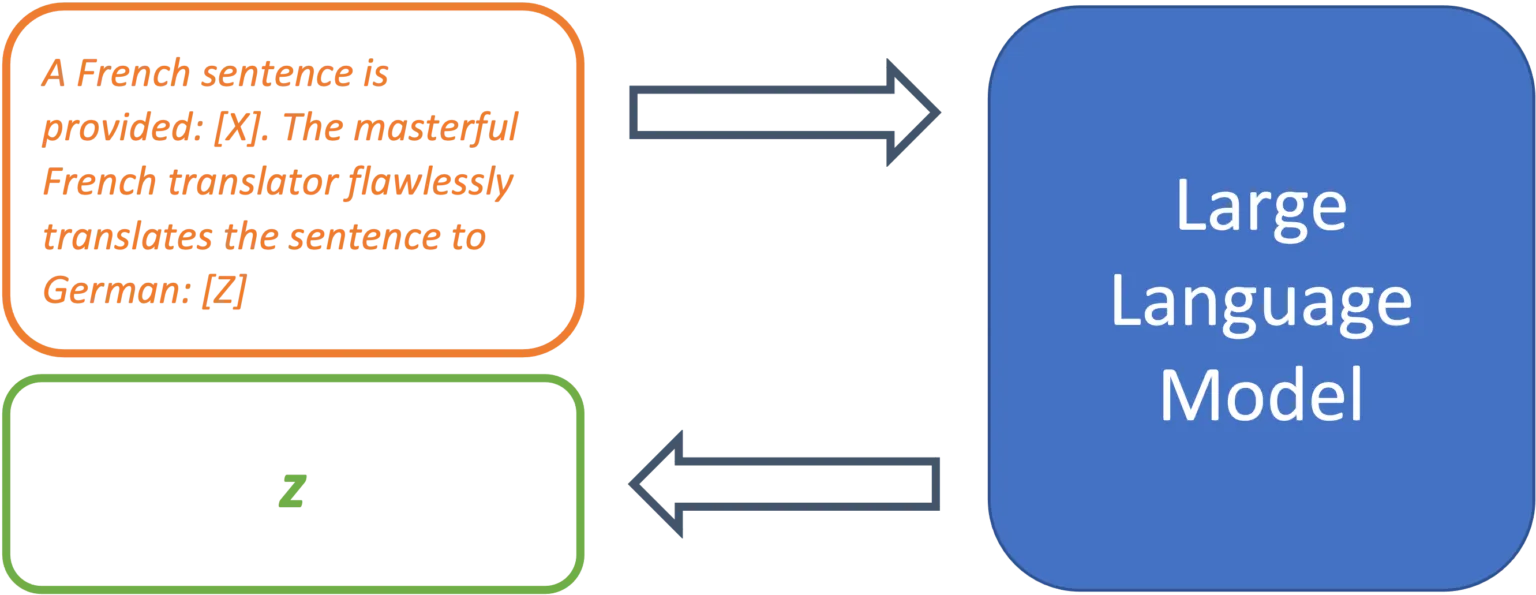
Figure 3: A clear task description can greatly increase the forecasting quality.
This is also recommended in use cases outside of translations. A text can be summarized with a simple command:
Summarize the following text: [X]: [Z]
However, better results can be expected with a more concrete prompt:
Rephrase this sentence with easy words so a child understands it,
emphasize practical applications and examples: [X]: [Z]
The more accurate the prompt, the greater the control over the output.
Prompts as constraints
Taken to its logical conclusion, the approach of controlling the model simply means constraining the model’s behavior through careful prompt design. This perspective is useful because during training, LLMs learn to complete many different sorts of texts and can thus solve a wide range of problems. With this design strategy, the basic approach to prompt design changes from describing the problem to excluding undesirable results by constraining model behavior. Which prompt leads to the desired result and only to the desired result? The following prompt indicates a translation task, but beyond that, it does not include any approaches to prevent the sentence from simply being continued into a story by the model.
Translate French to English Il pleut à Paris
One approach to improve this prompt is to use both semantic and syntactic means:
Translate this French sentence to English: “Il pleut à Paris.”
The use of syntactic elements such as the colon and quotation marks makes it clear where the sentence to be translated begins and ends. Also, the specification by sentence expresses that it is only about a single sentence. These measures reduce the likelihood that this prompt will be misunderstood and not treated as a translation problem.
Use of “memetic proxies”
This strategy can be used to increase the density of information in a prompt and avoid long descriptions through culturally understood context. Memetic proxies can be used in task descriptions and use implicitly understood situations or personae instead of detailed instructions:
A primary school teacher rephrases the following sentence: [X]: [Z]
This prompt is less descriptive than the previous example of rephrasing in simple words. However, the situation described contains a much higher density of information: The mentioning of an elementary school teacher already implies that the outcome should be understandable to children and thus hopefully increases the likelihood of practical examples in the output. Similarly, prompts can describe fictional conversations with well-known personalities so that the output reflects their worldview or way of speaking:
In this conversation, Yoda responds to the following question: [X]
Yoda: [Z]
This approach helps to keep prompts short by using implicitly understood context and to increase the information density within a prompt. Memetic proxies are also used in prompt design for other modalities. In image generation models such as DALL-e 2, the suffix “Trending on Artstation” often leads to higher quality results, although semantically no statements are made about the image to be generated.
Metaprompting
Metaprompting is how the research team of one study describes the approach of enriching prompts with instructions that are tailored to the task at hand. They describe this as a way to constrain a model with clearer instructions so that the task at hand can be better accomplished (Reynolds & McDonell, 2021). The following example can help to solve mathematical problems more reliably and to make the reasoning path comprehensible:
[X]. Let us solve this problem step-by-step: [Z]
Similarly, multiple choice questions can be enriched with metaprompts so that the model actually chooses an option in the output rather than continuing the list:
[X] in order to solve this problem, let us analyze each option and choose the best: [Z]
Metaprompts thus represent another means of constraining model behavior and results.

Figure 4: Metaprompts can be used to define procedures for solving problems.
Outlook
Prompt learning is a very young paradigm, and the closely related prompt engineering is still in its infancy. However, the importance of sound prompt writing skills will undoubtedly only increase. Not only language models such as GPT-3, but also the latest image generation models require their users to have solid prompt design skills in order to create convincing results. The strategies presented are both research and practice proven approaches to systematically writing prompts that are helpful for getting better results from large language models.
In a future blog post, we will use this experience with text generation to unlock best practices for another category of generative models: state-of-the-art diffusion models for image generation, such as DALL-e 2, Midjourney, and Stable Diffusion.
Sources
Brown, Tom B. et al. 2020. “Language Models Are Few-Shot Learners.” arXiv:2005.14165 [cs]. http://arxiv.org/abs/2005.14165 (March 16, 2022).
Reynolds, Laria, and Kyle McDonell. 2021. “Prompt Programming for Large Language Models: Beyond the Few-Shot Paradigm.” http://arxiv.org/abs/2102.07350 (July 1, 2022).