AI in the Workplace: How We Turn Skepticism into Confidence
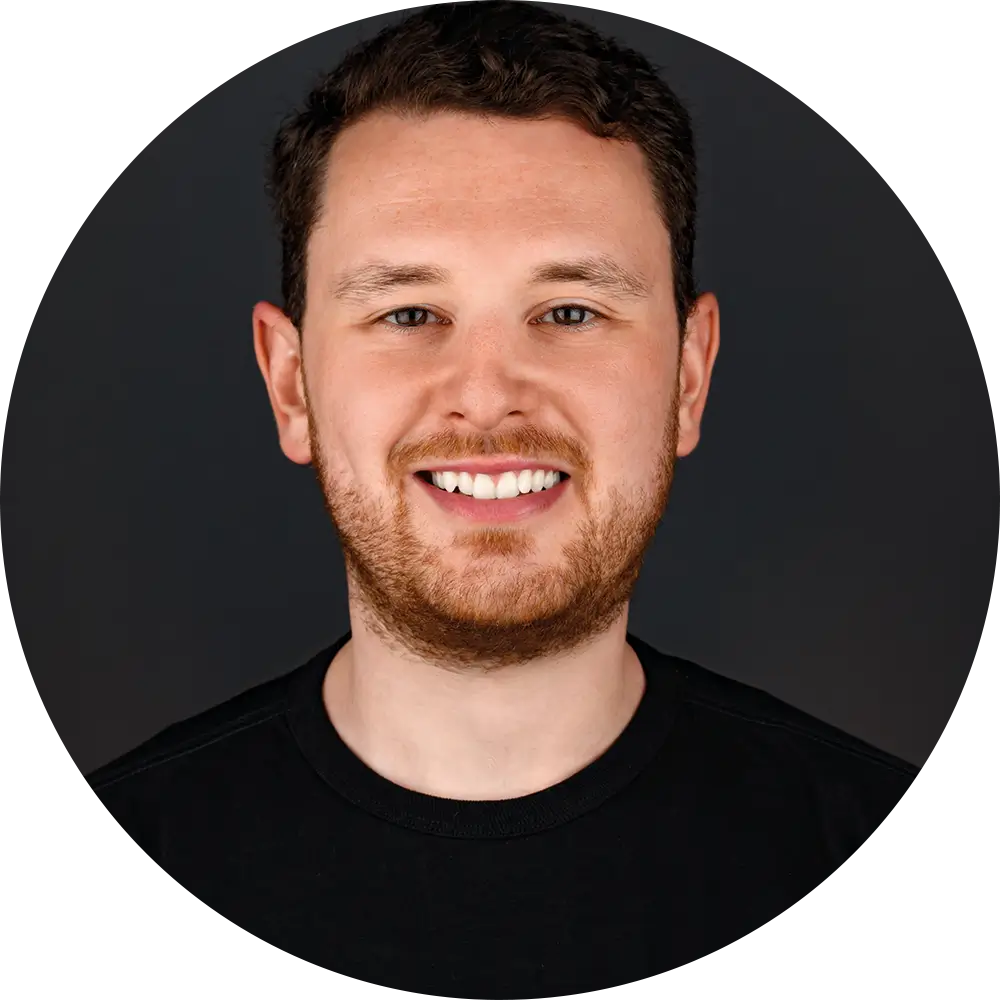

The discussion surrounding the use of artificial intelligence (AI) in the workplace appears to be splitting society. A tension is emerging: Some see AI as a groundbreaking advancement, while others fear it as a dystopian nightmare. There seems to be little middle ground.
At statworx, our AI & Society working group has made it its mission to dive deep into this discourse and find answers to the pressing questions facing society. To do so, we conducted a non-representative survey—partly online and partly in downtown Frankfurt—with 132 participants. We wanted to find out: What do people outside the AI bubble think about artificial intelligence in the workplace? Where do they see the greatest potential, and what are their biggest fears? Our goal was not just to collect opinions but to understand people’s fears and hopes in order to develop solutions for a socially responsible use of AI. To support our findings, we also analyzed other relevant studies and surveys and derived recommendations based on their insights.
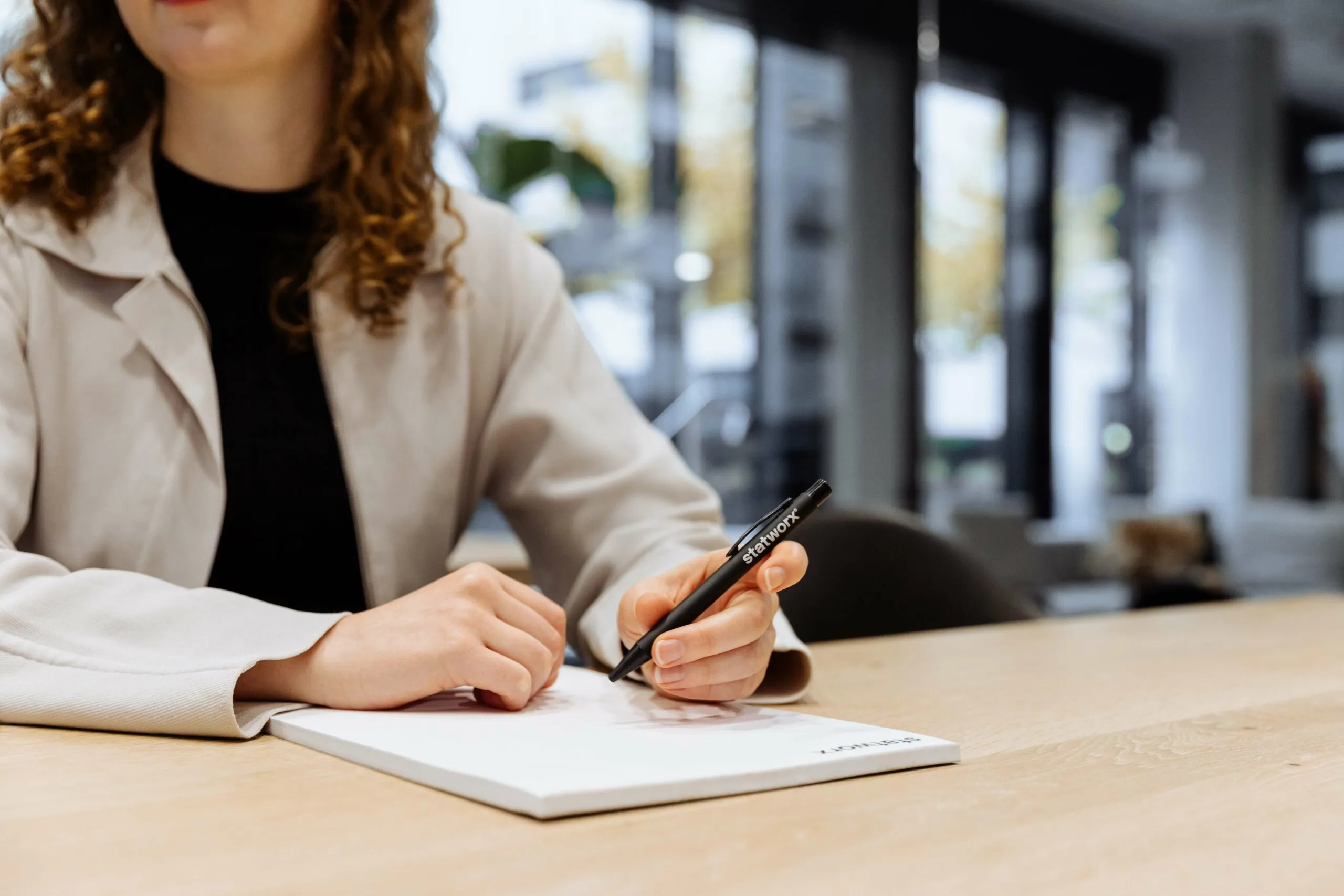
What People Think About AI
When it comes to the use of AI in the workplace, there is broad agreement on only one aspect: Companies and individuals who integrate AI into their daily work gain an advantage over others. Beyond that, opinions vary widely, creating a complex and sometimes contradictory picture drawn from studies, public sentiment, personal views, and emotions. Here are some key insights:
53% of our survey participants would like to use more AI applications in their studies and work. At the same time, 45% are unaware that they already use AI-powered services such as Google Maps and Spotify in their daily lives. This highlights a significant lack of awareness regarding what “artificial intelligence” actually includes—and what it does not.
Our survey also reveals that many people across different industries are worried about the future of AI. 55% say they are more concerned than excited about AI. More than half of the respondents even admitted to fearing the “general development” of AI. Similarly, 40% of respondents in a survey by the Allensbach Institute for Public Opinion Research said that generative AI unsettles them. Another study found that 58% of Germans consider AI “unappealing.” These results indicate that a significant portion of the population harbors vague fears and negative associations regarding AI.
When asked about AI’s impact on their own work, one-third of respondents expect a “rather strong” influence. However, nearly 60% believe AI will “have little or no effect” on their job. Less than a third expect AI to make their work more interesting, and only about a quarter believe AI will allow “more room for creativity.” These trends are supported by findings from an online survey conducted by the market research institute Bilendi, which focused on non-academic professionals with vocational training. One in four respondents stated that AI would primarily benefit companies, while employees themselves would not experience any real workload relief - instead, technological progress would simply increase the amount of work to be done. This suggests that AI’s impact on daily work is perceived more negatively than positively. However, only one-fifth of Bilendi’s respondents believe AI will eventually replace their job entirely. Likewise, 58% of our respondents do not believe AI will lead to higher unemployment, compared to 33% in the KIRA study, who worry about job losses.
What We Really Need to Prepare For
International comparison studies suggest that our survey respondents may be underestimating the impact of artificial intelligence. A 2023 Ipsos survey illustrates the low awareness in Germany regarding AI’s transformational potential:
- 35% of German respondents believe it is likely that AI will change their current job within the next five years (ranking second to last among surveyed countries, with an average of 57%).
- Only 19% think AI will replace their current job in the same timeframe, compared to a global average of 36%.
- Just 23% believe that the increased use of AI will improve their job in the next 3-5 years, while the international average is 37%.
- 40% expect AI to worsen the German labor market, while only 20% anticipate an improvement.
These results indicate that companies - and especially employees - feel unprepared for the challenges AI presents and lack a realistic understanding of the expected transformations. Educational opportunities and individual AI skills are lacking. These vague fears, combined with a lack of knowledge, are turning into concrete challenges for employers: How should companies handle differing employee perspectives on AI? How (if at all) should they address those who completely reject AI? And how should they manage those who are overly enthusiastic or even excessively eager? How can employees be empowered to use AI tools competently? Which departments and employees need which specific AI skills? And how should AI training be tailored to individual needs?
One thing is certain: Changes in generative AI are having a massive impact on the job market. On one hand, there is an increasing demand for professionals who combine data expertise with industry knowledge, leading to entirely new job roles, such as prompt engineers. On the other hand, AI-driven (partial) automation threatens mass layoffs in many industries. The recent announcement by BILD newspaper to cut staff due to ChatGPT is likely just a preview of what’s to come. Some estimates even suggest that up to 80% of jobs could be automated in the coming decades. The UN and other experts consider this unrealistic, but it underscores an important point: We don’t truly know where this journey will lead. This uncertainty arises because industries vary too greatly, job roles are multidimensional, and humans (for now) are not easily replaceable. Just because an AI system automates a specific task, it does not mean it can completely replace an entire job role. Even AI researchers agree: In a large-scale survey of over 2,700 AI experts, only 10% expected that AI could surpass humans in all tasks by 2027. However, half of the respondents believed that this technological breakthrough could happen by 2047. The only certainty is this: In the future, human workers will collaborate with AI across all industries.
Just a Lack of Knowledge? Understanding AI Skepticism
AI risks becoming a divisive societal issue if we fail to integrate it into the workplace in a socially responsible manner. How can we ensure that happens? Most studies point in the same direction: Education is a key factor in helping people understand AI’s legally permissible capabilities, make informed decisions about and with AI, and reduce fears surrounding the technology. Our survey confirms this: Even though they already use AI more frequently, executives still express a desire for more knowledge—as do 53% of respondents, who would like to use more AI in their professional environment. However, basic knowledge about the technology and its responsible use is not enough.
Many people also want to learn more about AI’s risks and find ways to empower themselves. This is strongly reflected in the qualitative responses from our study. One participant stated that “AI should be made understandable to all age groups so that no knowledge gap emerges.” Risk assessment is a critical issue for many, as another participant put it: “People are most interested in learning about AI risks—they are least interested in how AI actually works.” This heightened awareness of risk is also reflected in the KIRA study. In line with this, we found that when it comes to AI applications, respondents prioritize high security above all else, while fast availability is the least important factor. Interestingly, executives rate “high security” slightly lower and “fast availability” slightly higher than employees do.
In addition to concerns about direct AI risks, such as bias and discrimination, people also worry about dependency, loss of control, feeling overwhelmed, and fears of misuse or manipulation. A particularly prominent concern is AI-driven surveillance: 62% of our respondents and 54% of KIRA study participants share this fear. A similar trend appears with disinformation, which 51% of our respondents and 56% of KIRA participants associate with AI. Interestingly, however, 59% of our respondents do not believe AI poses a threat to humanity, whereas 58% of KIRA study participants are concerned about this possibility.
Who Are the AI Skeptics?
If the overwhelming amount of data and studies leaves you feeling uncertain, you are not alone. There is no clear picture. Even less can be inferred about how to specifically engage with AI skeptics. The discrepancies in responses reflect the complexity of AI perception and its impact on the labor market. The various perspectives and challenges highlight the need for a broad dialogue and participatory approach in shaping the future of AI in the workplace. But who do we need to engage with, and how?

A survey published in the MIT Sloan Management Review among 140 executives identifies three ideal types of AI-based decision-makers: skeptics, interactors, and delegators. Skeptics are unwilling to cede autonomy in decision-making to AI, while delegators are comfortable handing over responsibility to AI. Interactors take a middle path, adjusting their approach depending on the specific decision. The three decision-making styles reveal that the quality of an AI recommendation is only half of the equation when assessing AI-assisted decision-making in organizations. The human filter makes the difference, say Philip Meissner and Christoph Keding. Delegators tend to pass responsibility to others even without AI involvement.
A study by EY found that tech skeptics tend to be older, have lower incomes, and express dissatisfaction with their lives. They fear that future generations will be worse off and doubt that young people today will have a better life than their parents. Tech skeptics are concerned about their financial security, distrust the government, and are not convinced of technology’s benefits. While they use technology for basic tasks, they do not believe it will solve society’s problems. Although they possess fundamental digital skills, few see value in further developing them. Tech skeptics are generally opposed to data sharing, even when there is a clear purpose for it.
What Can We Learn from This?
Skepticism is a deeply human trait, often shaped by character and personality, and is not always receptive to purely logical arguments. Therefore, a cornerstone of any effort to address AI skepticism must be transparent, empathetic communication on equal footing that takes these concerns seriously.
A Forrester survey on AI in human resources identified four key groups that leaders should tailor their communication strategies to:
- AI Skeptics: Most commonly found in the IT sector
- AI Supporters: Typically between 26 and 35 years old and working in healthcare
- AI Indifferent: Most likely between 36 and 45 years old
- AI Enthusiasts: Often 18 to 25 years old, working in sales
Regardless of how well this classification applies to German society, creating personas can be a useful approach to developing appropriate messaging. Each of these groups reacts differently to various forms of communication. About half of AI supporters say that transparency regarding whether AI will lead to job losses in their own company would reduce their concerns and fears about AI in human resources. Only 18% of those who are indifferent to AI feel the same way. While more than half of AI skeptics stated that communication about how the company is using AI would alleviate their concerns and fears, only 22% of AI supporters share this view. 45% of cautious supporters and skeptics stated that they would be more likely to leave the company if their concerns about the use of AI in the HR department were not addressed.
How Can We Build AI Confidence?
We are still at the tip of the iceberg when it comes to AI adoption. As companies continue to expand their AI infrastructure, they must also ensure that their employees feel empowered to use AI in their respective roles. In other words, leadership must clearly demonstrate that they see their employees as partners, not just passengers, on the AI journey.

Our assessment is that more robust policies for responsible AI use in companies and teams are needed to turn concerns into confidence and prevent the unintended leakage of trade secrets or other sensitive data. This includes maximum transparency about the (planned) use of AI. The reality is that most employees do not truly understand how AI works, while many decision-makers believe they do. Upskilling helps bridge this gap. Targeted training for employees promotes a safe and productive adoption of AI. If employees also understand - because it is clearly demonstrated to them - how AI can improve their work, they will be more willing to embrace it. After all, most employees already hope that AI will help them access information more easily and increase their productivity. Even those who are skeptical are usually open to rational arguments. The key is how they are approached. Instead of assuming understanding and willingness, it can be helpful to engage them on an individual level: “When you have to make a major financial decision, do you rely solely on your gut feeling, or do you try to gather as much data and information as possible?” It must become clear that (in almost all typical cases) AI is a technology designed to help people make better, evidence-based decisions - not some dystopian scenario where humans become mere tools.
For this, targeted internal communication is important. Companies where tech and AI enthusiasts dominate may be best served by positioning AI as an exciting, revolutionary trend to their employees. If this audience values having a modern and high-tech employer, that should be reflected in communication. Other companies, where enthusiasm is lower and a more conservative, cautious mindset prevails, may find it more effective to present AI internally as a continuous improvement and evolution of existing, familiar technologies. They might even consider avoiding the AI label altogether and instead aligning new systems with familiar names and descriptions. There is no universal answer to which communication strategies work best in which company (and in which departments). However, the personas and their reasons for AI skepticism presented earlier provide valuable insights into how internal sentiment can be captured and used to develop an appropriate communication strategy.
About the AI & Society Working Group
As a working group with insights into the latest research, we engage in discussions with experts from business, society, and academia. Our work is not limited to analysis - we also take action. "KI Macht Schule" and Girls' Day are just a few examples of our efforts to involve society in the dialogue and make AI a tangible experience. The development of Responsible AI principles and workshops are additional measures aimed at driving innovation responsibly.
All results at a glance
Sources
- https://arxiv.org/pdf/2401.02843.pdf
- https://blog.workday.com/en-us/2023/how-employees-feel-ai-at-work.html
- https://www.elektroniknet.de/halbleiter/wer-hat-angst-vor-ki.207613.html
- https://www.ey.com/en_gl/insights/government-public-sector/meet-the-tech-skeptics
- https://www.faz.net/aktuell/politik/inland/allensbach-warum-deutsche-die-ki-fuerchten-19060081.html
- https://www.forbes.com/sites/joemckendrick/2021/10/18/skepticism-abounds-for-artificial-intelligence-in-high-level-decisions/
- https://www.ifd-allensbach.de/fileadmin/kurzberichte_dokumentationen/FAZ_Juli2023_KuenstlicheIntelligenz.pdf
- https://www.spiegel.de/karriere/homeoffice-viertagewoche-lebensarbeitszeit-welche-themen-beschaeftigte-2024-beschaeftigen-wird-a-7b7d1965-b75d-4a31-b094-dd850f4d04b9?giftToken=0f080248-2ed4-4439-899d-5e4881f21534&sara_ref=re-so-app-sh
- https://static1.squarespace.com/static/6426ad829db93559a3ed812e/t/64388f3a82787c5ebb290743/1681428318566/KIRA_Report_2023-4.pdf
- https://t3n.de/news/ki-prognosen-automatisierung-arbeit-1601598/
- https://www.un.org/en/desa/will-robots-and-ai-cause-mass-unemployment-not-necessarily-they-do-bring-other