Intro to Agentic AI and AI Agents
In this blog, we explore how AI agents are revolutionizing the work environment through autonomous and adaptive technologies, offering companies new opportunities for automation and efficiency enhancement.
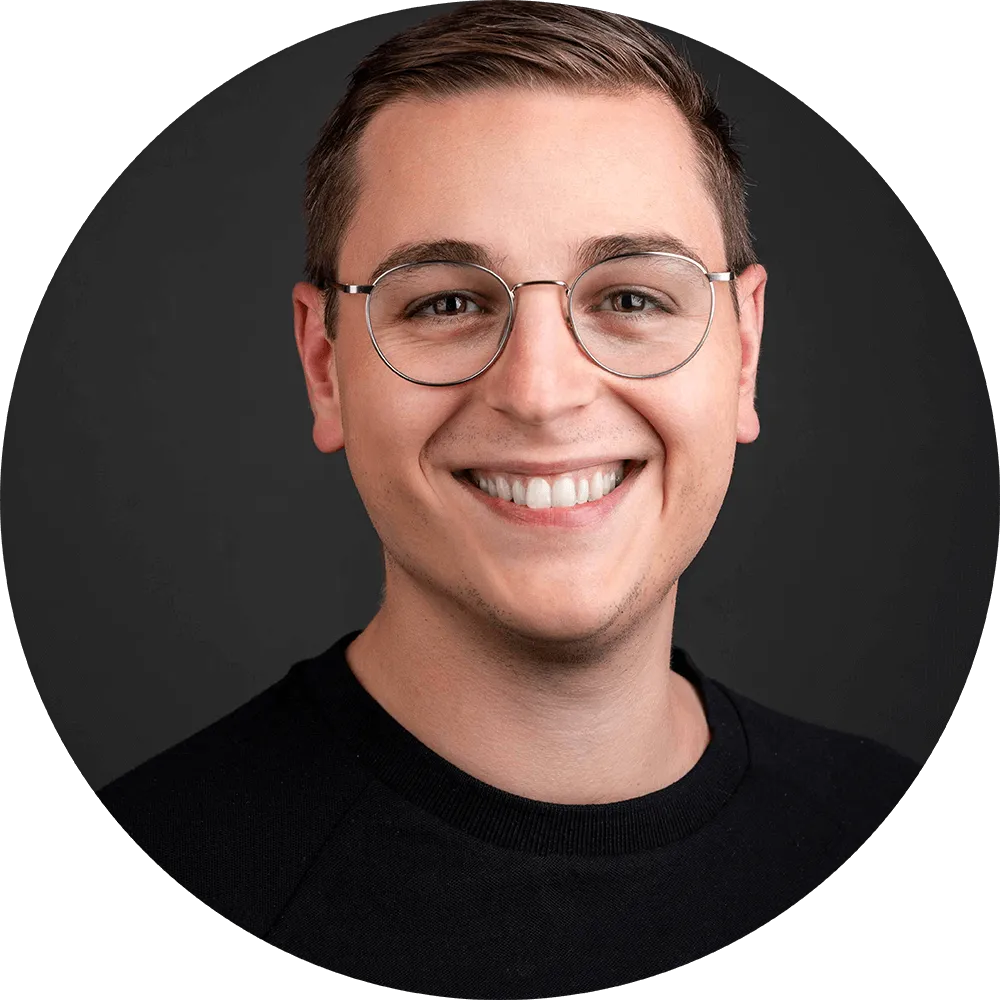
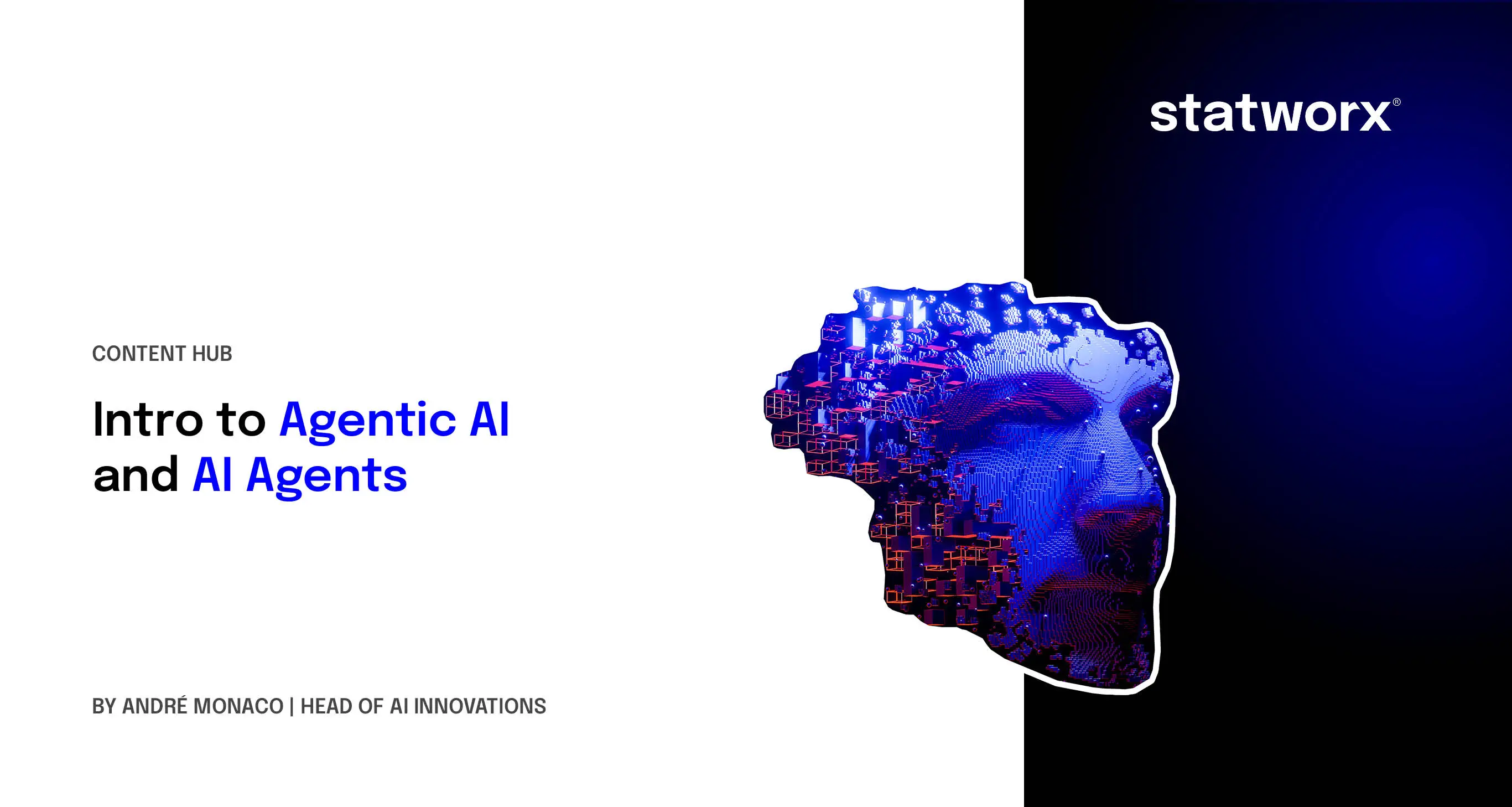
Artificial Intelligence has made significant strides in recent years. Following the hype surrounding Generative AI, the next evolution is on the horizon: AI Agents, also known as Agentic AI (in this article, we use these terms synonymously). Unlike current AI systems that merely respond to commands and queries, Agentic AI operates more dynamically. It not only reacts to commands and prompts with an answer – it adapts and can perform real actions such as sending emails, scheduling meetings, operating computers, and executing software code. This development opens up new possibilities, such as offering proactive, personalized services at scale for companies that essentially run themselves and save costs (e.g., negotiation agents in procurement) or optimize services and products (e.g., customer service agents).
But what exactly are AI Agents? This blog post delves into the origins, development, and examples to understand why organizations are heavily investing in them and how institutions can utilize this innovative technology. It highlights how they differ from previous AI systems and the transformative impact they have in various industries. Additionally, the article explores the current limitations of AI Agents and provides best-practice recommendations for dealing with them. Furthermore, we offer an overview of the various names and terminologies used to describe AI Agents, Agentic AI, or agent-based AI, AI assistants, and more.
Our goal is to clarify these terms and provide a comprehensive understanding of this advancing technology. We are currently experiencing the early iterations of Agentic AI in tools like ChatGPT and task automation software. And although these systems do not yet possess universal capabilities, they herald a future where Agentic AI will play an increasingly larger role in our daily lives. Apple Intelligence, for example, is still in its infancy but will soon harness the power of Agentic AI to control smartphones and other devices entirely through voice control.
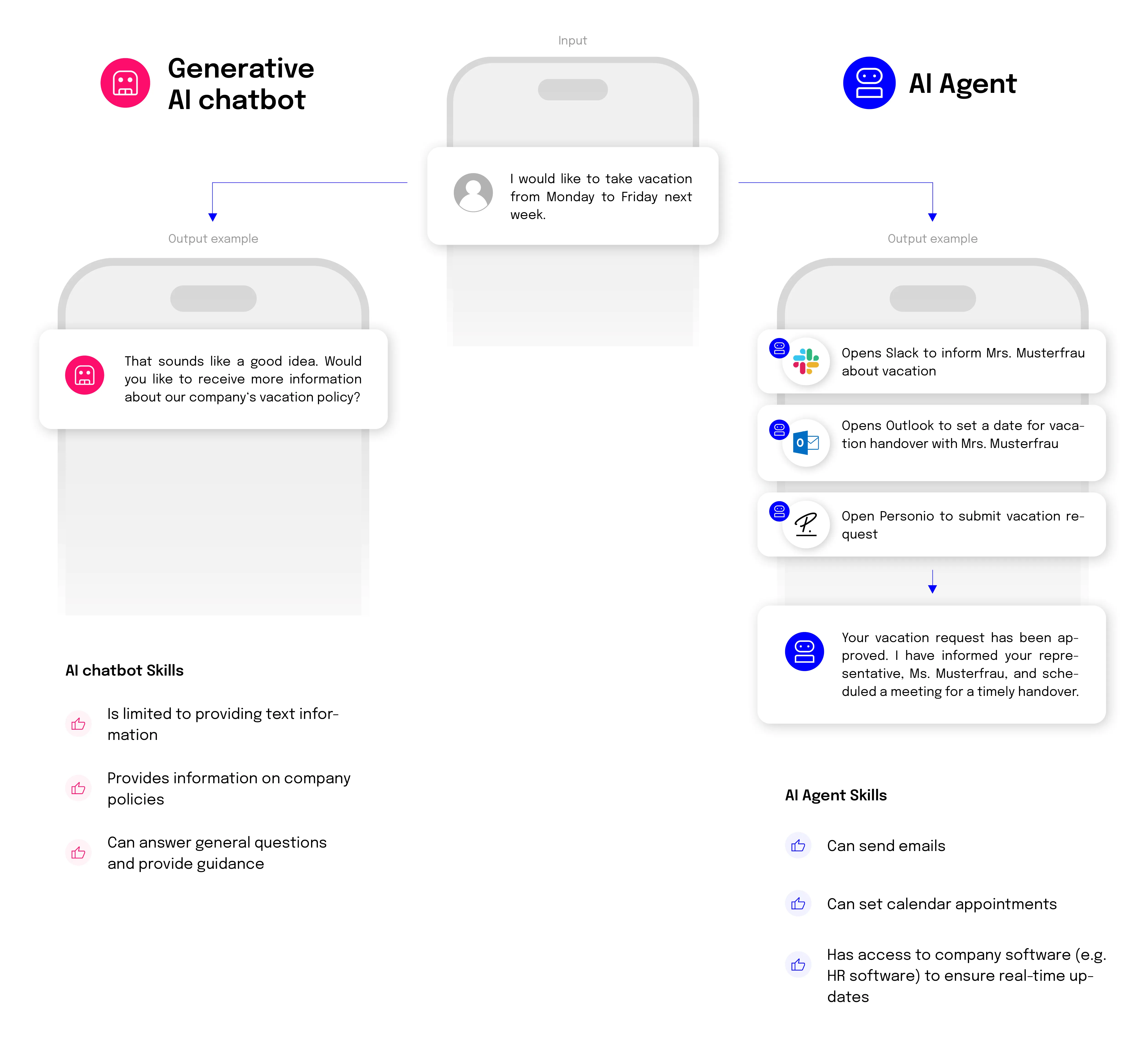
What is an AI Agent?
An AI Agent is like an advanced chatbot based on large language models. Unlike a regular chatbot, it plans the solution to tasks through an internal "monologue," communicating and "criticizing" itself. This results in more requests to the language model without the human noticing. Thus, the agent can independently interact with its environment, gather data, and perform tasks with minimal human intervention.
AI Agents can use tools and perform everyday tasks in the background, such as analyzing invoices and extracting data, while adapting to new information and dynamic environments. Agentic AI exists in two forms: software agents and embodied agents (simplified: robots). Both forms are based on advances in large language models (LLMs), enabling systems to autonomously perform complex tasks.
While software agents automate tasks like office work on digital platforms, embodied agents bring AI to life in 3D environments and robotics. They can, for example, make gaming experiences more realistic (Virtual & Augmented Reality), take over entire jobs (see https://www.figure.ai/, whose humanoid robots "work" at BMW in body construction), or revolutionize the working world as thinking, learning, and interacting humanoids.
How does an AI Agent work?
Perception & Information Gathering:
AI Agents process data from various sources, such as sensors, databases, and the internet, to extract meaningful features and obtain relevant information to execute planned tasks. The special feature: They can decide for themselves which tools they need for which task. Just as a new employee needs access to the company's internal software to work productively, an AI Agent also needs access to relevant interfaces. And just as you would show a new employee the intranet pages for the HR tool and all the other software with the note, "Read them at your leisure and contact us if you have questions," you simply give the AI Agent the documents to "read."
Planning, Goal Setting, Action:
The AI Agent receives a defined goal, plans the necessary tasks to make the result relevant and useful, and divides the goal into executable subtasks. Specifically, a language model coordinates various tools that generate solutions and autonomously execute tasks based on the plans. Safeguards ensure that tasks are carried out correctly.
Learning & Adaptation:
The continuous feedback loop enables the AI Agent to learn from data obtained from interactions. This leads to improved problem-solving ability, higher accuracy in decision-making, and increased efficiency in task execution.
A key feature of Agentic AI is the use of feedback mechanisms, such as interaction with other AI Agents and human intervention ("human in the loop," HITL). These mechanisms ensure iterative optimization of the AI Agent, as they can adapt and improve through communication, constructive criticism, and expert opinions—similar to humans. In this sense, AI Agents act like rational beings. They determine the most effective way to achieve a set goal by using everything available to them (data, humans, software, etc.) as a tool. It can also happen that an AI Agent turns to a human when it needs specific information to trigger a new action. It is also possible for an AI Agent to delegate a task back to a human if it cannot perform it independently. This means the autonomy of Agentic AI is expressed in its ability to interact with the environment, think, plan, and execute actions using its sensors and actuators. To prevent AI Agents from beginning to resist instructions and develop a life of their own, as suggested in some Hollywood productions, AI Safety and AI Alignment are crucial. Many companies and scientists insist that OpenAI and others consider security and alignment in development.
Instead, Agentic AI is designed to adapt to user expectations and provide personalized experiences, as well as comprehensive answers and actions. It can perform complex tasks by independently dividing them into subtasks, managing them, and correcting and updating itself when necessary. Unlike non-agentic chatbots, Agentic AI demonstrates a high degree of adaptability by evaluating its tools and effectively using available resources to bridge information gaps.

Overview of Terminologies
Various terms are swirling around in the public discourse on AI Agents. The concept of Agentic AI has its roots in the psychological principle of agency. Psychologist Albert Bandura brought human agency into the spotlight by examining how individuals consciously shape their actions and influence their environment. This research laid the foundation for the development of AI systems that aim to mimic these human-like abilities. With Agentic AI, we now have technology capable of thinking, learning, and acting. It can take proactive steps to tackle complex, multi-stage tasks and continuously improve through experience. This is fundamentally different from traditional AI systems and AI assistants.
- AI Agents / Agentic AI: Autonomous, learning systems capable of fulfilling tasks independently through perception of the environment, thinking, and acting to achieve specific goals. Related terms that capture (more or less) an aspect of this are:
- Autonomous Agents: Agents that operate independently without human intervention and make decisions based on their perceptions and goals.
- Virtual Agents: Software-based agents existing in virtual environments like video games or online platforms to fulfill tasks or interact with users.
- Embodied AI: AI systems that have a physical presence and perceive their environment through sensors and react or interact with it via actuators, such as robots.
- AI Collaboration & Co-Working / Human-AI Collaboration: These terms describe the collaborative integration of AI solutions with human teams to enhance creativity, productivity, and problem-solving capabilities. The goal here is to establish a symbiotic relationship where AI systems and humans collaborate and leverage each other's strengths to achieve superior results. This collaboration can occur with AI Agents (e.g., cursor.ai), but it can also occur with (currently) non-agentic AI tools like ChatGPT.
- AI Assistant: Virtual tools developed to support users in performing specific tasks, often using natural language processing for interaction. When referring to AI assistants, the human takes the lead in action and decision-making; the AI remains a tool with limited autonomy.
The 3 Components of an AI Agents
Architecture:
The architecture forms the foundation from which the agent operates. It can be a physical structure, a software program, or a combination of both. For example, a robotic AI Agent consists of actuators, sensors, motors, and robotic arms. An architecture hosting an AI software agent uses a text prompt, API, LLMs, and databases to enable autonomous operations.
Agent Function:
The agent function describes how the collected data is translated into actions that support the agent's goal. When designing the agent function, developers consider the type of information, AI capabilities, knowledge base, feedback mechanism, and other necessary technologies. In addition to providing access, it is only necessary to equip the agent with the required documentation on how tools can be used.
Agentenprogramm:
An agent program implements the agent function. It includes the development, training, and deployment of the AI Agent on the specified architecture. The agent program aligns the action logic, technical requirements, and performance elements of the agent. In short, this is where the agent is specified and equipped with the necessary agent functions.
Challenges and Best Practices in Dealing with Agentic AI
The development and use of AI Agents bring specific challenges ranging from privacy to technical limitations. To address these challenges, certain best practices are recommended.
In general, a gradual implementation of AI Agents is advisable, starting with pilot projects in controlled environments to test integration before widespread deployment. Robust monitoring should be established to continuously monitor the decisions of AI systems, ensuring transparency and accountability. A multi-layered security strategy is crucial to protect Agentic AI systems from potential threats. This strategy should include traditional cybersecurity measures as well as special precautions for AI, such as policy-based access controls, content monitoring, and real-time alerts for suspicious activities. Collaboration with AI and cybersecurity experts is also important to develop and refine integration strategies. Best practices should be adhered to, and a governance framework should be created.
Advantages Compared to Other AI Systems
AI Agents possess enormous potential, already causing significant changes in various sectors through automation and improved decision-making. Across industries, they transform organizations by converting large amounts of data into actionable knowledge and increasing employee efficiency. According to McKinsey, generative AI could automate up to 70% of worldwide work hours and increase corporate profits by $2.6 to $4.4 trillion annually. This shift allows human workers to focus on more strategic tasks and increase productivity.
- Productivity and Efficiency Improvement: Agentic AI automates repetitive and complex tasks, relieving human workers, minimizing errors, and reducing operational costs. By quickly processing large amounts of data and making decisions faster than human colleagues, significant time savings and increased operational efficiency are achieved.
- Scalability: The systems can handle numerous tasks simultaneously, making them ideal for scaling operations without increasing the need for additional human resources.
- Improved Decision-Making and Performance: With machine learning and real-time data processing, AI Agents offer well-founded analyzes and actionable insights. Multi-agent frameworks foster collaboration and knowledge exchange, enhancing decision-making and overall performance.
- Personalized Interactions and High-Quality Responses: Through real-time data analysis, Agentic AI creates tailored customer experiences and provides accurate, high-quality responses that enhance customer experience and satisfaction.
- Adaptability: Agentic AI can adapt to changing conditions and learn from new data, ensuring continuous improvement and relevance in evolving scenarios.
- Proactive Security: In cybersecurity, Agentic AI can proactively identify and mitigate threats, reducing response times and minimizing potential damage.
Impacts on Various Industries
AI Agents will massively influence almost all industries due to their ability to efficiently automate tasks and offer personalized services. According to LangChains State of AI Agents Report, the adoption of AI Agents is increasing across all industries: 51% of respondents (over 1,300 professionals from engineers and product managers to CEOs and executives) already use them, and 78% plan to implement them soon. Regarding the type of tasks, AI Agents are mainly used for research and summaries (58%), personal productivity (53.5%), and customer service (45.8%).
The introduction is hindered by difficulties developers face when explaining the capabilities and behaviors of agents to various stakeholders in the company. Complex AI systems are black boxes, and their actions are not always explainable. Therefore, companies proceed cautiously and ensure robust controls are in place to effectively regulate agent behavior. But already today, AI Agents deliver significant benefits in some industries:
In customer service, they automatically process inquiries, provide immediate solutions, and personalized recommendations, enhancing the overall experience and enabling 24/7 support. Retailers use AI Agents for personalized shopping experiences and optimizing inventory and logistics. Notably, there is interest in "Digital Humans" that embody brands and provide lifelike interactions during high call volumes.
In healthcare, AI Agents support real-time patient monitoring, provide critical data during surgical procedures, plan treatments, and efficiently distribute resources, improving patient care and operational efficiency.
In finance, AI Agents optimize trading, fraud detection, credit collection, and risk management processes, making financial services faster and more accurate. They also act as virtual financial advisors, offering individual investment suggestions based on a customer’s financial history and goals. They independently identify cross-selling and upselling opportunities, anticipate customer needs, and proactively offer relevant information. Their ability for autonomous fraud detection allows them to analyze transaction patterns, flag suspicious behavior, and react immediately.
In the transport sector, AI Agents improve traffic management systems and optimize routes for autonomous vehicles, leading to safer and more efficient transportation networks.
In cybersecurity, AI Agents can be used for automated threat detection and response by identifying threats in real-time and reacting to them. For example, an AI-powered security system can detect unusual network activities and autonomously isolate affected devices to prevent security incidents without the need for human intervention. Additionally, Agentic AI can be used for network optimization by efficiently managing network traffic and ensuring that critical applications receive the necessary resources while simultaneously minimizing bottlenecks and potential security risks.
Examples of AI Agents
Specialized software agents
General agent architectures
Conclusion & Outlook
Agentic AI has the potential not only to realize efficiency gains but also to fundamentally change the way companies operate. Employees can focus on strategic tasks while repetitive activities are taken over by AI. AI agents work autonomously and are highly capable of learning, enabling them to perform complex tasks independently and flexibly adapt to new information. This development marks the next big step towards a fully automated and intelligent corporate world. In the future, specialized AI agents could emerge that address industry-specific needs and collaborate in multi-agent systems, similar to human teams. Additionally, AI as a Service (AIAaaS) could facilitate companies' access to advanced AI.
The direction of this journey will be significantly influenced by the major players in Silicon Valley. However, companies can already take action now by developing implementation strategies, training their employees, and creating prototypes. Statworx offers a comprehensive package for companies, ranging from workshops for executives and developers to their own AI agent prototypes. This enables companies to strategically transition from rigid RPA processes to AI agents, thereby increasing their efficiency and capacity for innovation.