How to Get Your Data Science Project Ready for the Cloud

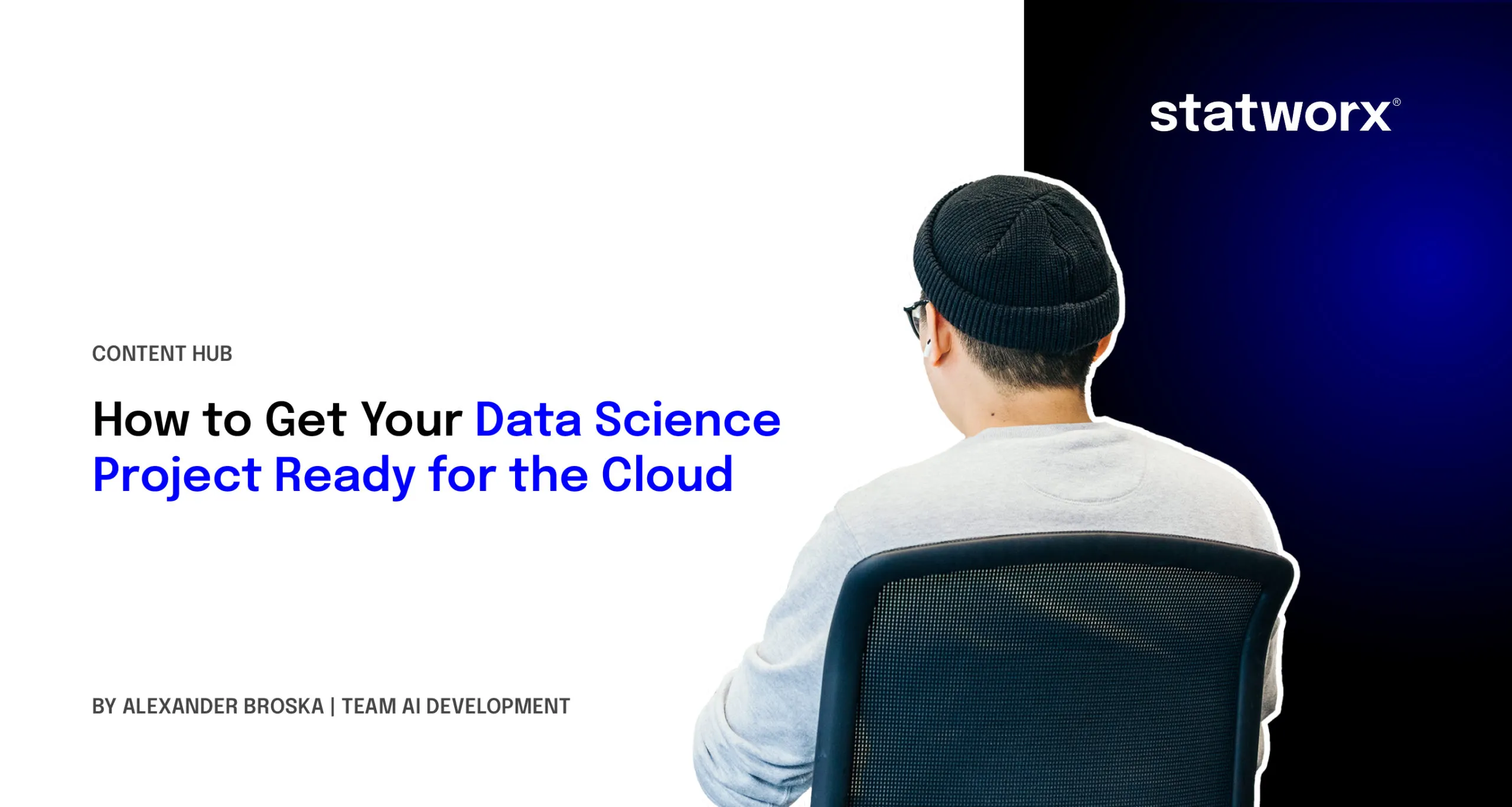
Create more value from your projects with the cloud.
Data science and data-driven decision-making have become a crucial part of many companies’ daily business and will become even more important in the upcoming years. Many organizations will have a cloud strategy in place by the end of 2022:
“70% of organizations will have a formal cloud strategy by 2022, and the ones that fail to adopt it will struggle”
– Gartner Research
By becoming a standard building block in all kinds of organizations, cloud technologies are getting more easily available, lowering the entry barrier for developing cloud-native applications.
In this blog entry, we will have a look at why the cloud is a good idea for data science projects. I will provide a high-level overview of the steps needed to be taken to onboard a data science project to the cloud and share some best practices from my experience to avoid common pitfalls.
I will not discuss solution patterns specific to a single cloud provider, compare them or go into detail about machine learning operations and DevOps best practices.
Data Science projects benefit from using public cloud services
One common approach to data science projects is to start by coding on local machines, crunching data, training, and snapshot-based model evaluation. This helps keeping pace at an early stage when not yet confident machine learning can solve the topic identified by the business. After having created the first version satisfying the business needs, the question arises of how to deploy that model to generate value.
Running a machine learning model in production usually can be achieved by either of two options: 1) run the model on some on-premises infrastructure. 2) run the model in a cloud environment with a cloud provider of your choice. Deploying the model on-premises might sound appealing at first and there are cases where it is a viable option. However, the cost of building and maintaining a data science specific piece of infrastructure can be quite high. This results from diverse requirements ranging from specific hardware, over managing peak loads in training phases up to additional interdependent software components.
Different cloud set-ups offer varying degrees of freedom
When using the cloud, you can choose the most suitable service level between Infrastructure as a Service (IaaS), Container as a Service (CaaS), Platform as a Service (PaaS) and Software as a Service (SaaS), usually trading off flexibility for ease of maintenance. The following picture visualizes the responsibilities in each of the service levels.
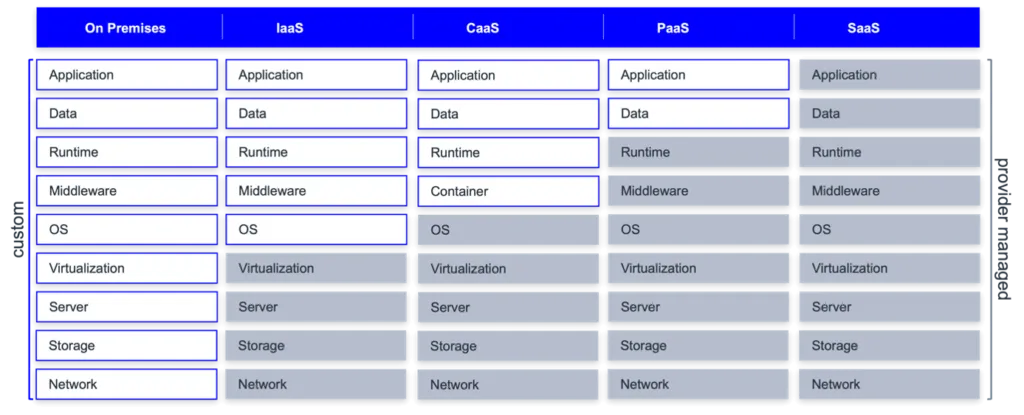
- «On-Premises» you must take care of everything yourself: ordering and setting up the necessary hardware, setting up your data pipeline and developing, running, and monitoring your applications.
- In «IaaS» the provider takes care of the hardware components and delivers a virtual machine with a fixed version of an operating system (OS).
- With «CaaS» the provider offers a container platform and orchestration solution. You can use container images from a public registry, customize them or build your own container.
- With «PaaS» services what is usually left to do is bring your data and start developing your application. Depending on whether the solution is serverless you might not even have to provide information on the sizing.
- «SaaS» solutions as the highest service level are tailored to a specific purpose and include very little effort for setup and maintenance, but offer quite limited flexibility for new features have usually to be requested from the provider
Public cloud services are already tailored to the needs of data science projects
The benefits of public cloud services include scalability, decoupling of resources and pay-as-you-go models. Those benefits are already a plus for data science applications, e.g., for scaling resources for a training run. On top of that, all 3 major cloud providers have a part of their service catalog designed specifically for data science applications, each of them with its own strengths and weaknesses.
Not only does this include special hardware like GPUs, but also integrated solutions for ML operations like automated deployments, model registries and monitoring of model performance and data drift. Many new features are constantly developed and made available. To keep up with those innovations and functionalities on-premises you would have to spend a substantial number of resources without generating direct business impact.
If you are interested in an in-depth discussion of the importance of the cloud for the success of AI projects, be sure to take a look at the white paper published on our content hub.
Onboarding your project to the cloud takes only 5 simple steps
If you are looking to get started with using the cloud for data science projects, there are a few key decisions and steps you will have to make in advance. We will take a closer look at each of those.
1. Choosing the cloud service level
BWhen choosing the service level, the most common patterns for data science applications are CaaS or PaaS. The reason is that infrastructure as a service can create high costs resulting from maintaining virtual machines or building up scalability across VMs. SaaS services on the other hand are already tailored to a specific business problem and are used instead of building your own model and application.

CaaS comes with the main advantage of containers, namely that containers can be deployed to any container platform of any provider. Also, when the application does not only consist of the machine learning model but needs additional micro-services or front-end components, they can all be hosted with CaaS. The downside is that similar to an on-premises roll-out, Container images for MLops tools like model registry, pipelines and model performance monitoring are not available out of the box and need to be built and integrated with the application. The larger the number of used tools and libraries, the higher the likelihood that at some point future versions will have incompatibilities or even not match at all.
PaaS services like Azure Machine Learning, Google Vertex AI or Amazon SageMaker on the other hand have all those functionalities built in. The downside of these services is that they all come with complex cost structures and are specific to the respective cloud provider. Depending on the project requirements the PaaS services may in some special cases feel too restrictive.
When comparing CaaS and PaaS it mostly comes down to the tradeoff between flexibility and a higher level of vendor lock-in. Higher vendor lock-in comes with paying an extra premium for the sake of included features, increased compatibility and rise in the speed of development. Higher flexibility on the other hand comes at the cost of increased integration and maintenance effort.
2. Making your data available in cloud
Usually, the first step to making your data available is to upload a snapshot of the data to a cloud object storage. These are well integrated with other services and can later be replaced by a more suitable data storage solution with little effort. Once the results from the machine learning model are suitable from a business perspective, data engineers should set up a process to automatically keep your data up to date.
3. Building a pipeline for preprocessing
In any data science project, one crucial step is building a robust pipeline for data preprocessing. This ensures your data is clean and ready for modeling, which will save you time and effort in the long run. A best practice is to set up a continuous integration and continuous delivery (CICD) pipeline to automate deployment and testing of your preprocessing and to make it part of your DevOps cycle. The cloud helps you automatically scale your pipelines to deal with any amount of data needed for the training of your model.
4. Training and evaluating trained models
In this stage, the preprocessing pipeline is extended by adding modeling components. This includes hyper-parameter tuning which cloud services once again support by scaling resources and storing the results of each training experiment for easier comparison. All cloud providers offer an automated machine learning service. This can be used either to generate the first version of a model quickly and compare performance on the data across multiple model types. This way you can quickly assess if the data and preprocessing suffice to tackle the business problem. Besides that, the result can be utilized as a benchmark for the data scientist. The best model should be stored in a model registry for deployment and transparency.
In case a model has already been trained locally or on-premises, it is possible to skip the training and just load the model into the model registry.
5. Serving models to business users
The final and likely most important step is serving the model to your business unit to create value from it. All cloud providers offer solutions to deploy the model in a scalable manner with little effort. Finally, all pieces created in the earlier steps from automatically provisioning the most recent data over applying preprocessing and feeding the data into the deployed model come together.
Now we have gone through the steps of how to onboard your data science project. With these 5 steps you are well on the way with moving your data science workflow to the cloud. To avoid some of the common pitfalls, here are some learnings from my personal experiences I would like to share, which can positively impact your project’s success.
Make your move to the cloud even easier with these useful tips
Start using the cloud early in the process.
By starting early, the team can familiarize themselves with the platform’s features. This will help you make the most of its capabilities and avoid potential problems and heavy refactoring down the road
Make sure your data is accessible.
This may seem like a no-brainer, but it is important to make sure your data is easily accessible when you move to the cloud. This is especially true in a setup where your data is generated on-premises and needs to be transferred to the cloud.
Consider using serverless computing.
Serverless computing is a great option for data science projects because it allows you to scale your resources up or down as needed without having to provision or manage any servers.
Don’t forget about security.
While all cloud providers offer some of the most up-to-date IT-security setups, some of them are easy to miss during configuration and can expose your project to needless risk.
Monitor your cloud expenses.
Coming from on-premises, optimization is often about peak resource usage because hardware or licenses are limited. With scalability and pay-as-you-go, this paradigm shifts stronger towards optimizing costs. Optimizing costs is usually not the first activity to do when starting a project but keeping an eye on the costs can prevent unpleasant surprises and be used at a later stage to make a cloud application even more cost-effective.
Take your data science projects to new heights with the cloud
If you’re starting your next data science project, doing so into the cloud is a great option. It is scalable, flexible, and offers a variety of services that can help you get the most out of your project. Cloud based architectures are a modern way of developing applications, that are expected to grow even more important in the future.
Following the steps presented will help you on that journey and support you in keeping up with the newest trends and developments. Plus, with the tips provided, you can avoid many of the common pitfalls that may occur on the way. So, if you’re looking for a way to get the most out of your data science project, the cloud is definitely worth considering.