Master R shiny: One trick to build maintainable and scaleable event chains
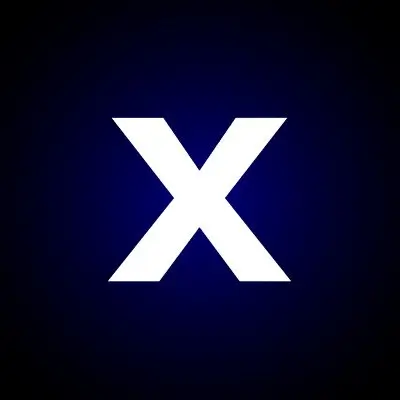
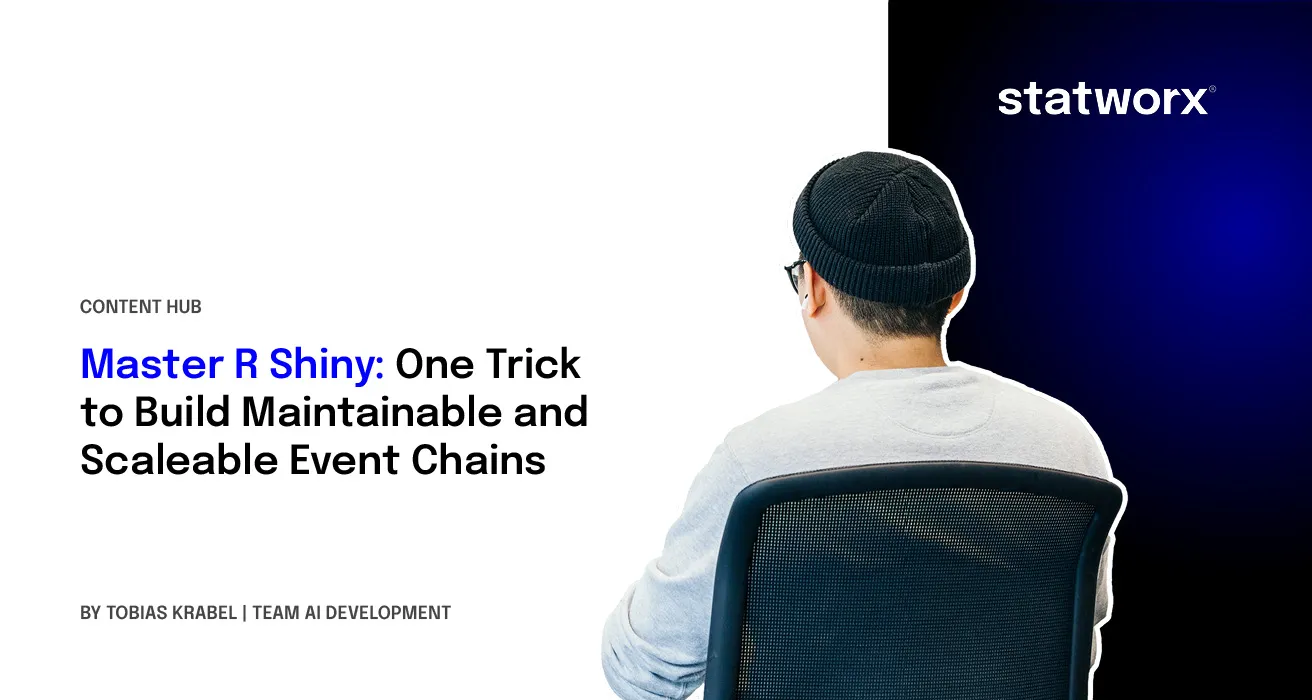
Introduction
Writing appealing interactive web applications – one of STATWORX’s many competencies – is easy with R shiny. Just a few lines of code in one R script create the whole logic you need to let the whole magic of shiny happen. It is so simple that you can make a hello world app in a heartbeat, like so.
library(shiny)
ui <- fluidPage(
"Hello, World!"
)
server <- function(input, output, session) { }
shinyApp(ui, server)
Today I am going to show you one way you can use native shiny syntax to modularize pieces of your code in a way that makes your code basis easily maintainable and extendable. Since I assume you are already familiar with shiny, I’ll skip the intro wading pool example and go right to the high-dive.
What are event chains?
An event chain describes the relationship between events and tasks and how the events affect each other. In some cases, you may want to have an app that takes user input and performs actions based on the nature of the input, potentially asking for more information along the way. In such a case, chances are you want to implement an event chain. You could immediately start hacking some crude solution to your problem, but you may risk creating hardly comprehensible code. Furthermore, imagine that requirements on your event chain suddenly change. In this case, it is important to modularize your event chain so that it remains maintainable and adaptable.
Example: the friend logger
So, let me illustrate how to build a modularized event chain. Imagine you are pedantic about time and take appointments seriously. Quite to the detriment of your so-called “friends”, you make no exceptions. Every time a friend is too late, you suffer so bad you have decided to use a shiny app to keep score of your friends’ visits in order to determine how reliable they are (you pathetic you!). Requirements for the app’s usage are simple, as shown in the graph below.
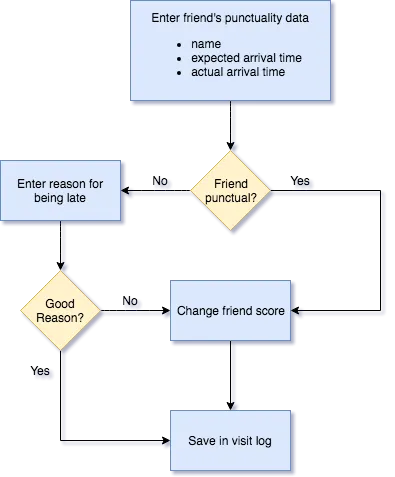
You want to compare the expected arrival time of your friend with his actual arrival time. If his delay is above a certain threshold (e.g. 5 minutes), you want to protocol his excuse for being late. If you deem his excuse as being acceptable, you neglect his sin (but still keep protocol!). If he is punctual, he receives a bonus point. If he arrives too late and his excuse is not acceptable, he receives a minus point. In any case, you log his visit (how low can you get?). To keep things more visual, here is a sketch of the app’s UI including the event sequence when a friend is being late.
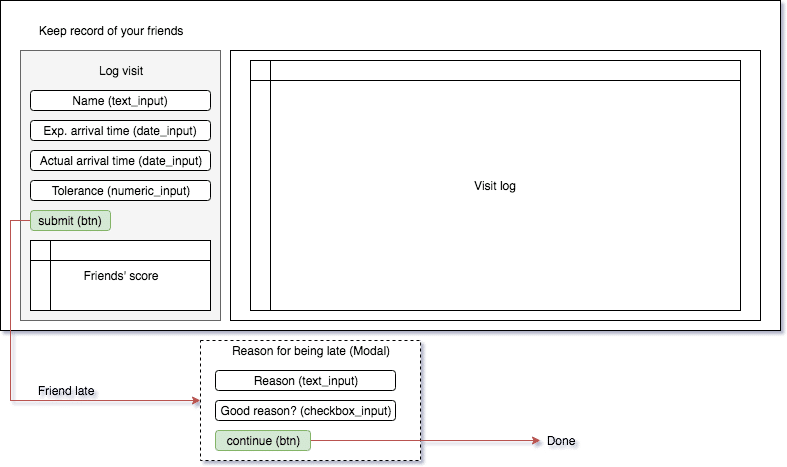
Now, it is time to implement the app.
Event chain architecture in R Shiny
It takes two ingredients to implement event chains:
- triggers that are stored in
reactiveValues()
- observers (
observeEvent()
) that are triggered and carry out the actual checks and other computations
The actual trick is to find the appropriate number of observeEvent()
s so that each step in the event chain is covered by one observeEvent
and therefore no code redundancies are created. Using the example above, we have three possible sequences of events:
- Friend is too late and has a good excuse
- Friend is too late and doesn’t have a good excuse
- Friend is not too late
In all three cases, we need to log a friend’s visit, so it definitely makes sense to put the visit logging part in one observeEvent
and to call that observer at the end of each of the sequences above. Drawing an event chain diagram comes in especially handy here, as it supports a suitable architectural design choice. I used draw.io for the task.
For the app, I used one reactiveValues
-object in which I put all triggers (you can find the whole app code on GitHub).
shinyServer(function(input, output, session) {
# Data
rv <- reactiveValues(
...
# Triggers
ask_for_reason = TRUE,
change_friend_score = TRUE,
save_visit = TRUE,
error = FALSE
)
...
})
I use boolean values for the triggers so that I only have to negate them if I want to change their value (a <- !a
). Using integers would also work, but I find the flip-trick nicer. Let’s look at the part of the chain where a friend’s punctuality is checked in more detail. The module that checks punctuality also reads in the data. Depending on the input, it either calls the “Ask-for-a-reason”-module or directly calls the visit logger.
# Submit friend data ----
observeEvent(input(dollar sign)submit, {
# Collect data
...
is_delayed <- difftime(actual_time, expected_time, units = "mins") > input(dollar sign)acceptance
if (is_delayed) {
# Friend is delayed --> trigger Ask-for-reason-module
rv(dollar sign)ask_for_reason <- isolate(!rv(dollar sign)ask_for_reason)
return()
}
# Friend seems punctual --> Add a point to score list :)
friend_data <- set_data(friend_data, score = 1)
# Trigger visit logger
rv(dollar sign)change_friend_score <- isolate(!rv(dollar sign)change_friend_score)
})
As you can see, once you have drawn the event chain it is quite intuitive to translate it into shiny code. If the friend is punctual, we set his score to one (score
will be added in the visit logger module) and call the visit logger module, which looks like this:
# Change friend score ----
observeEvent(rv(dollar sign)change_friend_score, ignoreInit = TRUE, {
rv(dollar sign)friend_score[friend_score(dollar sign)name == friend_data(dollar sign)name, "score"] <-
isolate(rv(dollar sign)friend_score[friend_score(dollar sign)name == friend_data(dollar sign)name, "score"]) +
friend_data(dollar sign)score
# Make change permanent
saveRDS(rv(dollar sign)friend_score, "data/friend_score.RDS")
rv(dollar sign)save_visit <- isolate(!rv(dollar sign)save_visit)
})
Note that the rv(dollar sign)save_visit
trigger simply calls an observer that adds another row to the friend visit table and does some cleaning.
So now let’s make a little test run with the ready product. For your app to work, you of course have to first create an initial dataset with your friends and their initial scores in order to know who you are keeping a record of. In the example below, we have four friends: John, Emily, Olivia, and Ethan. You can see their scores in the lower-left corner. Past visits are logged and displayed in the upper right corner.
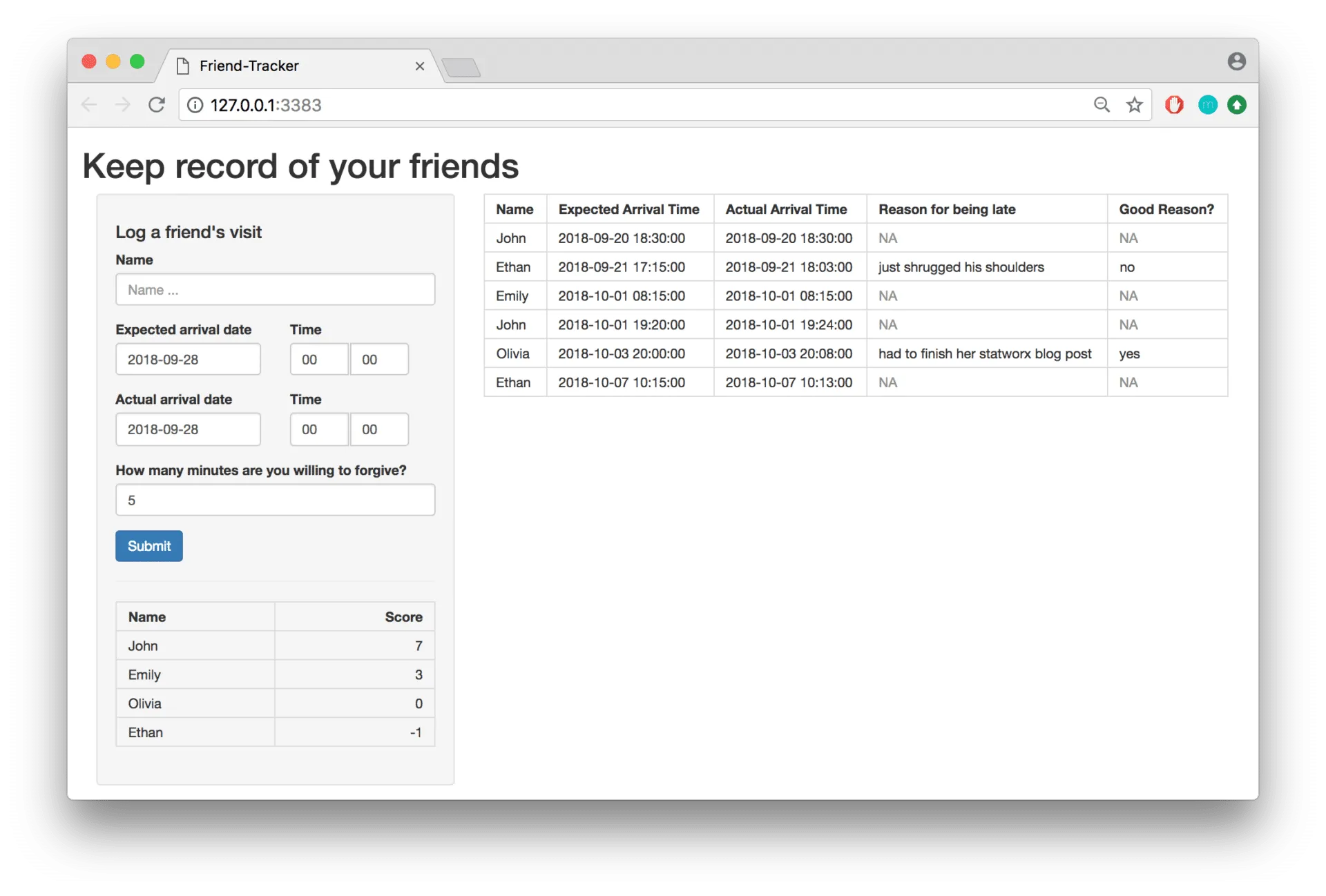
John wants to hang out with us to play some brutal video games, and for no obvious reason, we made an appointment at 9 am. However, John shows up 7 (!!!) minutes late. Enough is enough. We enter his misdeed.
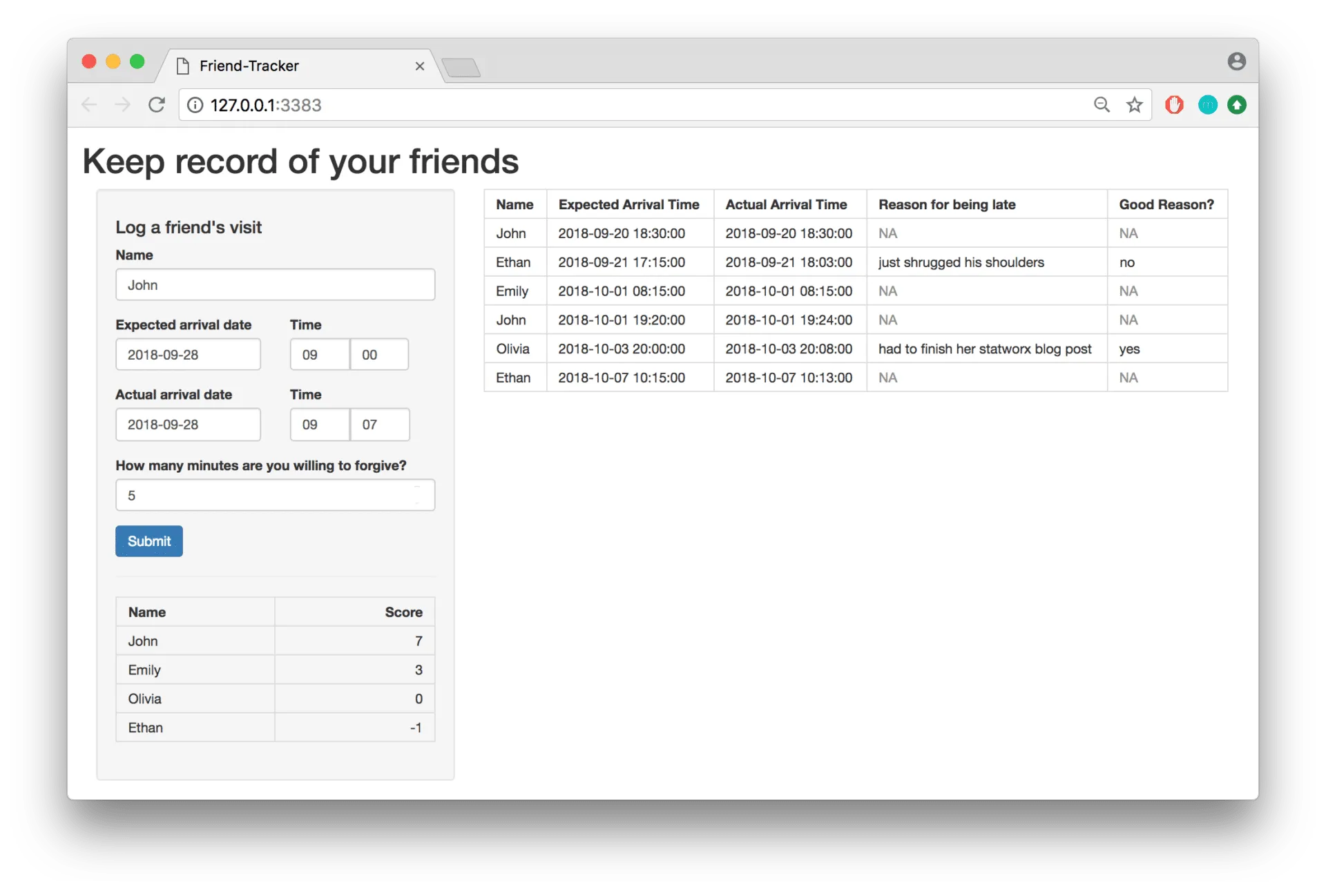
It exceeds the threshold, so we are, as expected, prompted to enter the reason.

When we asked John to justify himself, he just shrugged his shoulders. How dare he?! That’s a minus point…
Extend our event chain
Even though you are hurt because of John’s unreliability, you are quite happy with your app. Yet, things could be better! For example, every time you misspell a friend in the name field when protocoling a visit, the app crashes. Your app could use some (additional) sanity checks. A perfect use case for showing the flexibility of your architecture. After a few months of deep reflection, you came up with a new event flow graph that takes care of wrong inputs.

You figured two spots where the app ought to be stabilized. First, you want to throw an error to the user if a friend doesn’t exist (without stopping the app). Second, you require yourself to enter a reason (we all know how sloppy our future self can be from time to time).
With the already chosen modularized structure, it is easy to incorporate these checks. You simply need to add one more trigger (rv(dollar sign)error
) and one global container that stores the error information.
# Error handler
error <- reactiveValues(
title = "",
message = ""
)
If you for example want to check whether an entered name exists in your database, all you have to do is to add a few lines of code at the beginning of the observer where a friend’s punctuality is checked.
# Submit friend data ----
observeEvent(input(dollar sign)submit, {
# Friend exists?
if (!input(dollar sign)name %in% rv(dollar sign)friend_score(dollar sign)name) {
error(dollar sign)title <- "%s not found" %>% sprintf(., input(dollar sign)name)
error(dollar sign)message <- h1("404")
rv(dollar sign)error <- isolate(!rv(dollar sign)error)
return()
}
...
})
If the name doesn’t match any of your friends’ names, you trigger an error handler module whose only purpose is to show an error message:
# Error handling ----
observeEvent(rv(dollar sign)error, ignoreInit = TRUE, {
showModal(modalDialog(
title = error(dollar sign)title,
error(dollar sign)message,
footer = actionButton("exit", "Ok", class = "btn-primary")
))
})
The nice thing is that you can use this module to handle any errors, no matter which sanity checks have caused them.
So if we go back to the app now and enter a name that doesn’t exist (like Tobias), we get the following error message:
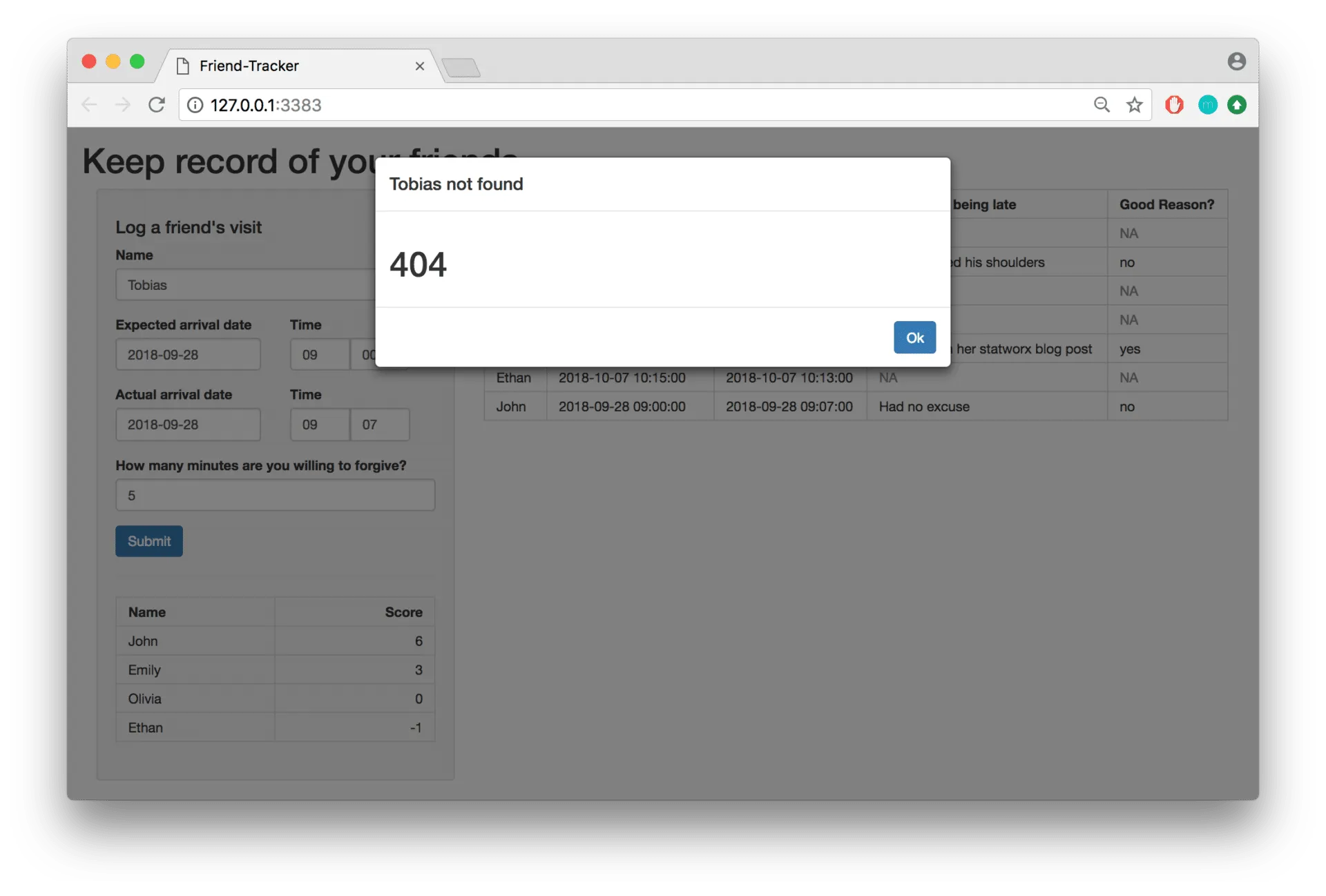
Furthermore, if we miss entering a reason when being asked for one, we get a passive-aggressive reminder:

You are welcome! So would you excuse me now? I have some visits to protocol…