Introduction to non-parametric statistics: The Wilcoxon sign test
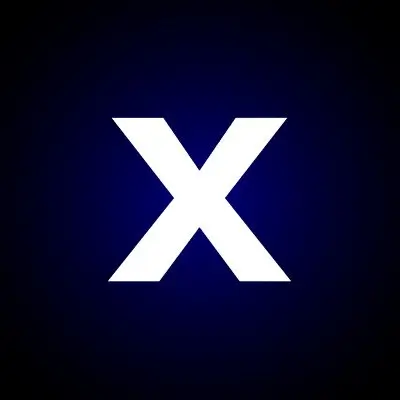
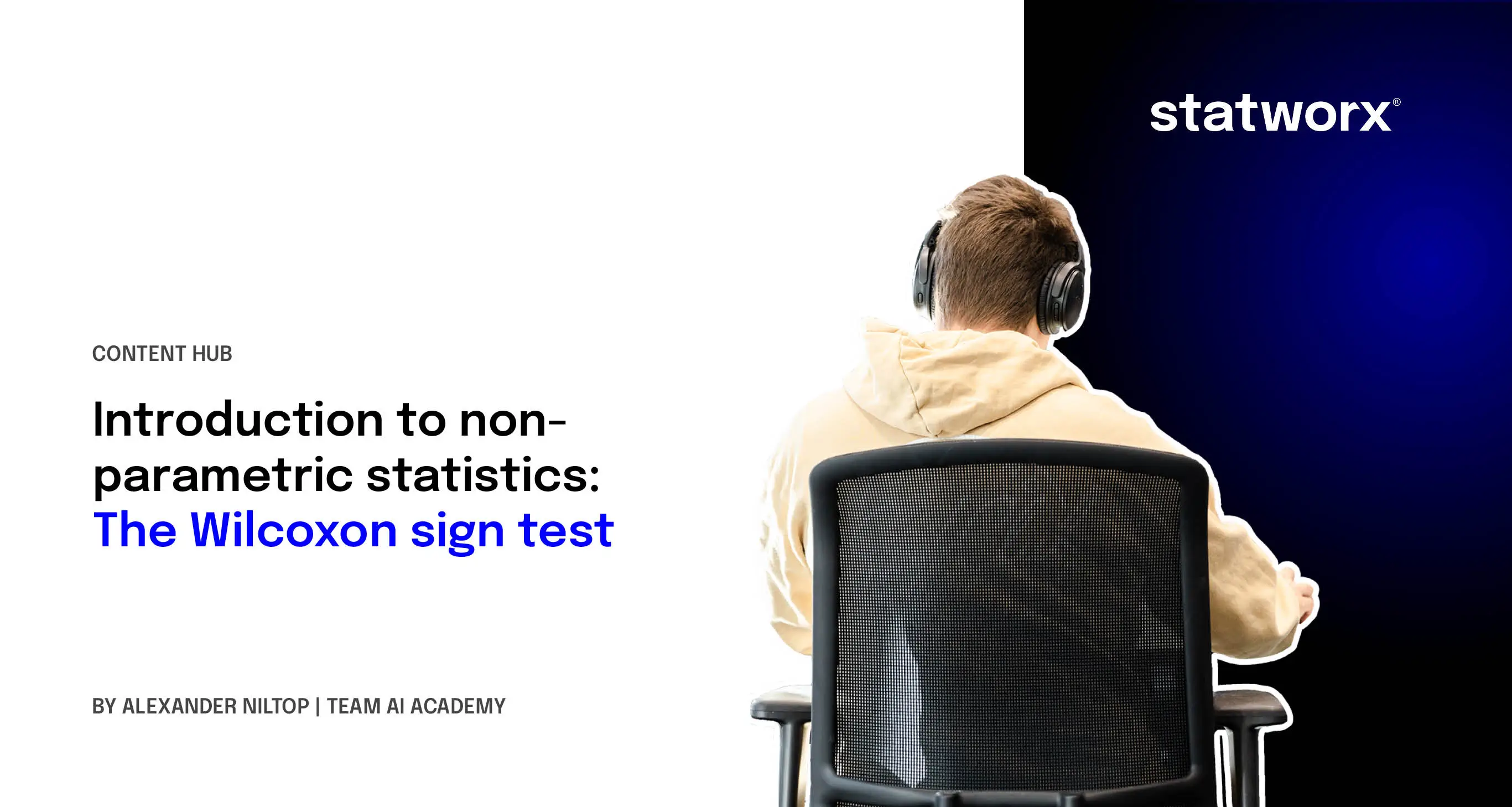
Although we narrowly escaped the pull of the black hole last time, which lies near the world of the Wilcoxon rank-sum test (The full story is here!), we are not intimidated and continue our journey of discovery through the non-parametric universe.
The next planet we want to explore at first glance could be mistaken for the world of the Wilcoxon rank-sum test (More interested in that? Then click here.). Both worlds have similar flora and fauna, but upon closer inspection, there are small yet significant differences. Acissej, our onboard botanist and feared throughout the universe by the big-footed blue-foreheaded leaf hens, is an expert in this field. She will therefore lead our expedition to explore the world of the Wilcoxon signed-rank test.
Two worlds – Similarities and differences
The first thing that becomes apparent during the exploration is a similarity between the two worlds: The Wilcoxon signed-rank test and the Wilcoxon rank-sum test are each the non-parametric alternative to the t-test. The former is the counterpart to the t-test for dependent samples, and the latter to the t-test for independent samples (What is a t-test? Find out here.). Non-parametric means that both tests make less strict assumptions about the distribution of the dependent variable. Additionally, both share the characteristic of testing the medians of two groups for significant differences. Acissej jokingly adds that the inventor of both tests – Frank Wilcoxon – has immortalized himself in the name of each.
Despite the many similarities between the two worlds, there is one major difference: the situation in which the application of the test is appropriate. The Wilcoxon signed-rank test is used exclusively for dependent samples. But what exactly does dependence mean? Often it means that a particular characteristic was measured twice in different individuals. In this case, it’s called repeated measurement. Dependence can also mean that the values of two people can be linked through a common factor. Acissej immediately thinks of a good example: If two members of our crew have to share a cabin and one is in a bad mood, you immediately know how the other is doing. Whether they like it or not, their moods are dependent on each other! However, for dependencies between measurements, only those that show a certain systematic pattern can be considered(1). For example, the mood of one crew member could influence the mood of all members on board. But since it is not (easily) measurable who talks to whom and thus influences each other, this is a form of dependency that would not be statistically accounted for.
The depths of the world of the Wilcoxon sign test
Acissej is in an extremely good mood today and has the perfect example ready to explain the detailed nature of the world of the Wilcoxon signed-rank test. To do so, she pulls a note from her pocket, on which we can read the following:
Her example, of course, involves plants. Acissej conducted a small experiment and now wants to know whether it was successful. Yesterday, she planted 15 new plants in her cabin and measured their height. However, instead of using regular soil, she used coffee grounds for planting. She got the idea when she recently saw on another world that the locals there grow plants on the remains of other plants. Since she knows as a botanist how many nutrients are in coffee and she herself drinks at least 10 cups a day, using coffee grounds seemed like a brilliant idea. This morning, she took new measurements and noted everything down on her sheet. Now she wants to know whether the plants have grown on average so that she doesn't torment them if the whole thing was a silly idea.
Since “unfortunately” only 15 plants fit in her cabin and the measurements are dependent, the Wilcoxon signed-rank test is recommended. This test first calculates the differences between the two time points: size at planting – size the next day (see Table 2). Then, for each difference, the sign is recorded and ranks are assigned. When assigning the ranks, the sign is ignored (see Table 2).
The ranks are ultimately summed into two rank sums: one rank sum for ranks with positive signs (T+) and one for ranks with negative signs (T-). To test whether the medians in both groups differ, the test uses the rank sum of the positive differences T+. In our case, this is 11. The corresponding p-value for T+ is then calculated using one of two methods. Either by standardizing the rank sum relative to the number of individuals in the group and dividing by its standard error, or by calculating the p-value exactly using a simulation. Since each value in the data appears only once and the sample size is smaller than 40, the exact method must be applied. The exact p-value for the plant heights is 0.003, and the result is therefore significant. One can thus assume that the median plant height after one day (20.81) is significantly greater than at the time of planting (20.35). So not only have Acissej’s plants grown on average, but we can also assume that coffee grounds are suitable as a growing medium for other plants as well. To celebrate, Acissej grabs herself a coffee!
References
- Eid, M., Gollwitzer, M. & Schmitt, M. (2015). Statistik und Forschungsmethoden (4. Überarbeitete und erweiterte Auflage). Weinheim: Beltz. S. 368.
- Wilcoxon, F. (1945). Individual comparisons by ranking methods. Biometrics, 1, 80-83.