Break the Bias in AI
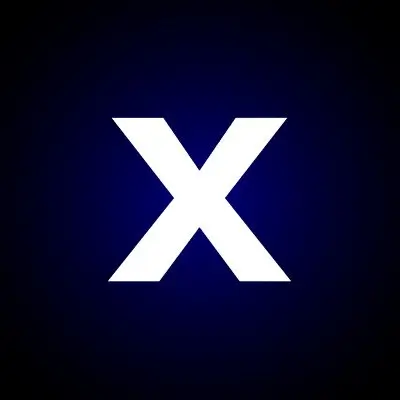
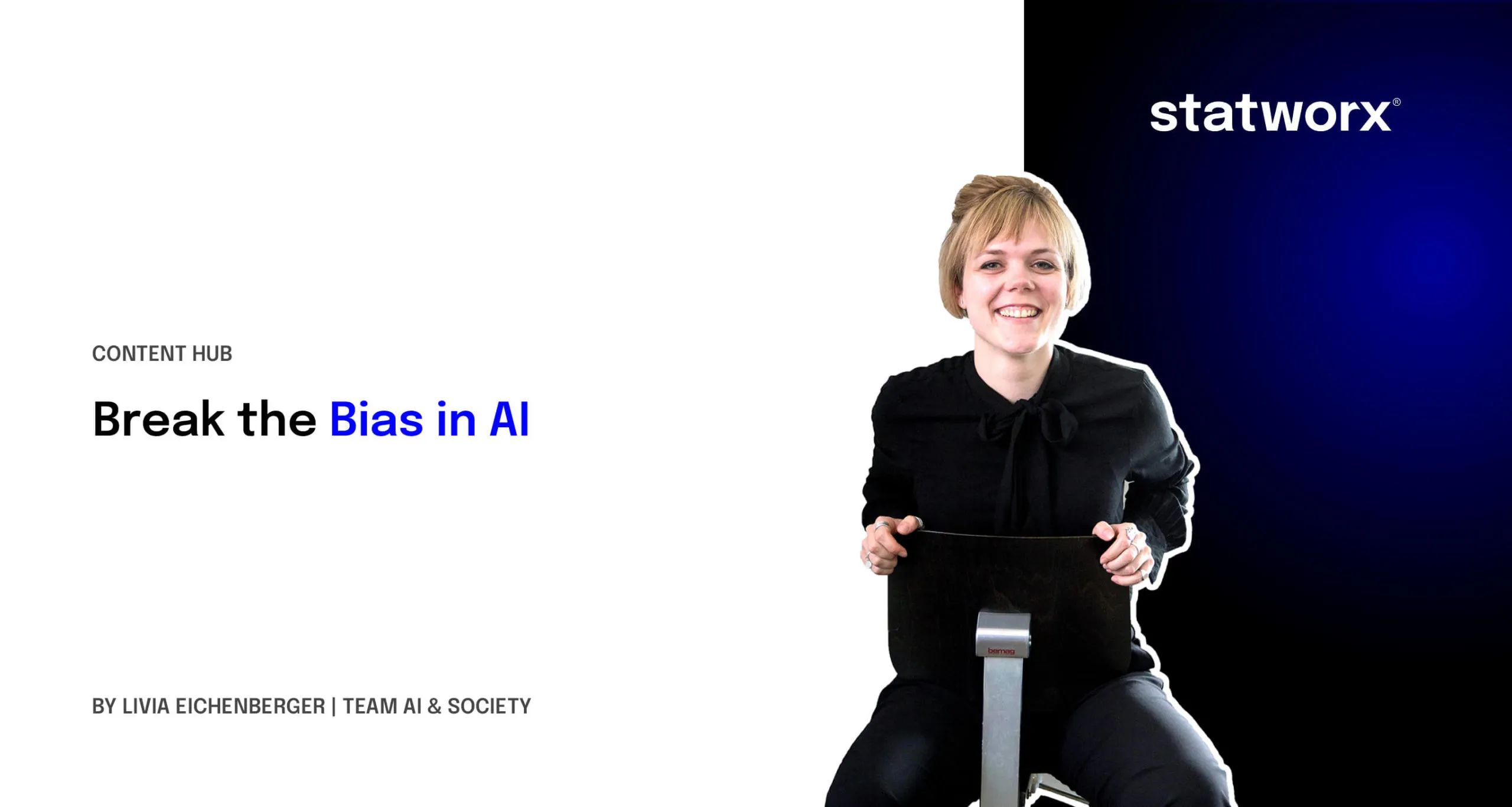
Whether deliberate or unconscious, bias in our society makes it difficult to create a gender-equal world free of stereotypes and discrimination. Unfortunately, this gender bias creeps into AI technologies, which are rapidly advancing in all aspects of our daily lives and will transform our society as we have never seen before. Therefore, creating fair and unbiased AI systems is imperative for a diverse, equitable, and inclusive future. It is crucial not only to be aware of this issue but that we act now, before these technologies reinforce our gender bias even more, including in areas of our lives where we have already eliminated them.
Solving starts with understanding: To work on solutions to eliminate gender bias and all other forms of bias in AI, we first need to understand what it is and where it comes from. Therefore, in the following, I will first introduce some examples of gender-biased AI technologies and then give you a structured overview of the different reasons for bias in AI. I will present the actions needed towards fairer and more unbiased AI systems in a second step.
Sexist AI
Gender bias in AI has many faces and has severe implications for women’s equality. While Youtube shows my single friend (male, 28) advertisements for the latest technical inventions or the newest car models, I, also single and 28, have to endure advertisements for fertility or pregnancy tests. But AI is not only used to make decisions about which products we buy or which series we want to watch next. AI systems are also being used to decide whether or not you get a job interview, how much you pay for your car insurance, how good your credit score is, or even what medical treatment you will get. And this is where bias in such systems really starts to become dangerous.
In 2015, for example, Amazon’s recruiting tool falsely learned that men are better programmers than women, thus, not rating candidates for software developer jobs and other technical posts in a gender-neutral way.
In 2019, a couple applied for the same credit card. Although the wife had a slightly better credit score and the same income, expenses, and debts as her husband, the credit card company set her credit card limit much lower, which the customer service of the credit card company could not explain.
If these sexist decisions were made by humans, we would be outraged. Fortunately, there are laws and regulations against sexist behavior for us humans. Still, AI has somehow become above the law because an assumably rational machine made the decision. So, how can an assumably rational machine become biased, prejudiced, and racist? There are three interlinked reasons for bias in AI: data, models, and community.

Data is Destiny
First, data is a mirror of our society, with all our values, assumptions, and, unfortunately, also biases. There is no such thing as neutral or raw data. Data is always generated, measured, and collected by humans. Data has always been produced through cultural operations and shaped into cultural categories. For example, most demographic data is labeled based on simplified, binary female-male categories. When gender classification conflates gender in this way, data is unable to show gender fluidity and one’s gender identity. Also, race is a social construct, a classification system invented by us humans a long time ago to define physical differences between people, which is still present in data.
The underlying mathematical algorithm in AI systems is not sexist itself. AI learns from data with all its potential gender biases. For example, suppose a face recognition model has never seen a transgender or non-binary person because there was no such picture in the data set. In that case, it will not correctly classify a transgender or non-binary person (selection bias).
Or, as in the case of Google translate, the phrase “eine Ärztin” (a female doctor) is consistently translated into the masculine form in gender-inflected languages because the AI system has been trained on thousands of online texts where the male form of “doctor” was more prevalent due to historical and social circumstances (historical bias). According to Invisible Women, there is a big gender gap in Big Data in general, to the detriment of women. So if we do not pay attention to what data we feed these algorithms, they will take over the gender gap in the data, leading to serious discrimination of women.
Models need Education
Second, our AI models are unfortunately not smart enough to overcome the biases in the data. Because current AI models only analyze correlations and not causal structures, they blindly learn what is in the data. These algorithms inherent a systematical structural conservatism, as they are designed to reproduce given patterns in the data.
To illustrate this, I will use a fictional and very simplified example: Imagine a very stereotypical data set with many pictures of women in kitchens and men in cars. Based on these pictures, an image classification algorithm has to learn to predict the gender of a person in a picture. Due to the data selection, there is a high correlation between kitchens and women and between cars and men in the data set – a higher correlation than between some characteristic gender features and the respective gender. As the model cannot identify causal structures (what are gender-specific features), it thus falsely learns that having a kitchen in the picture also implies having women in the picture and the same for cars and men. As a result, if there’s a woman in a car in some image, the AI would identify the person as a man and vice versa.
However, this is not the only reason AI systems cannot overcome bias in data. It is also because we do not “tell” the systems that they should watch out for this. AI algorithms learn by optimizing a certain objective or goal defined by the developers. Usually, this performance measure is an overall accuracy metric, not including any ethical or fairness constraints. It is as if a child was to learn to get as much money as possible without any additional constraints such as suffering consequences from stealing, exploiting, or deceiving. If we want AI systems to learn that gender bias is wrong, we have to incorporate this into their training and performance evaluation.
Community lacks Diversity
Last, it is the developing community who directly or indirectly, consciously or subconsciously introduces their own gender and other biases into AI technologies. They choose the data, define the optimization goal, and shape the usage of AI.
While there may be malicious intent in some cases, I would argue that developers often bring their own biases into AI systems at an unconscious level. We all suffer from unconscious biases, that is, unconscious errors in thinking that arise from problems related to memory, attention, and other mental mistakes. In other words, these biases result from the effort to simplify the incredibly complex world in which we live.
For example, it is easier for our brain to apply stereotypic thinking, that is, perceiving ideas about a person based on what people from a similar group might “typically “be like (e.g., a man is more suited to a CEO position) than to gather all the information to fully understand a person and their characteristics. Or, according to the affinity bias, we like people most who look and think like us, which is also a simplified way of understanding and categorizing the people around us.
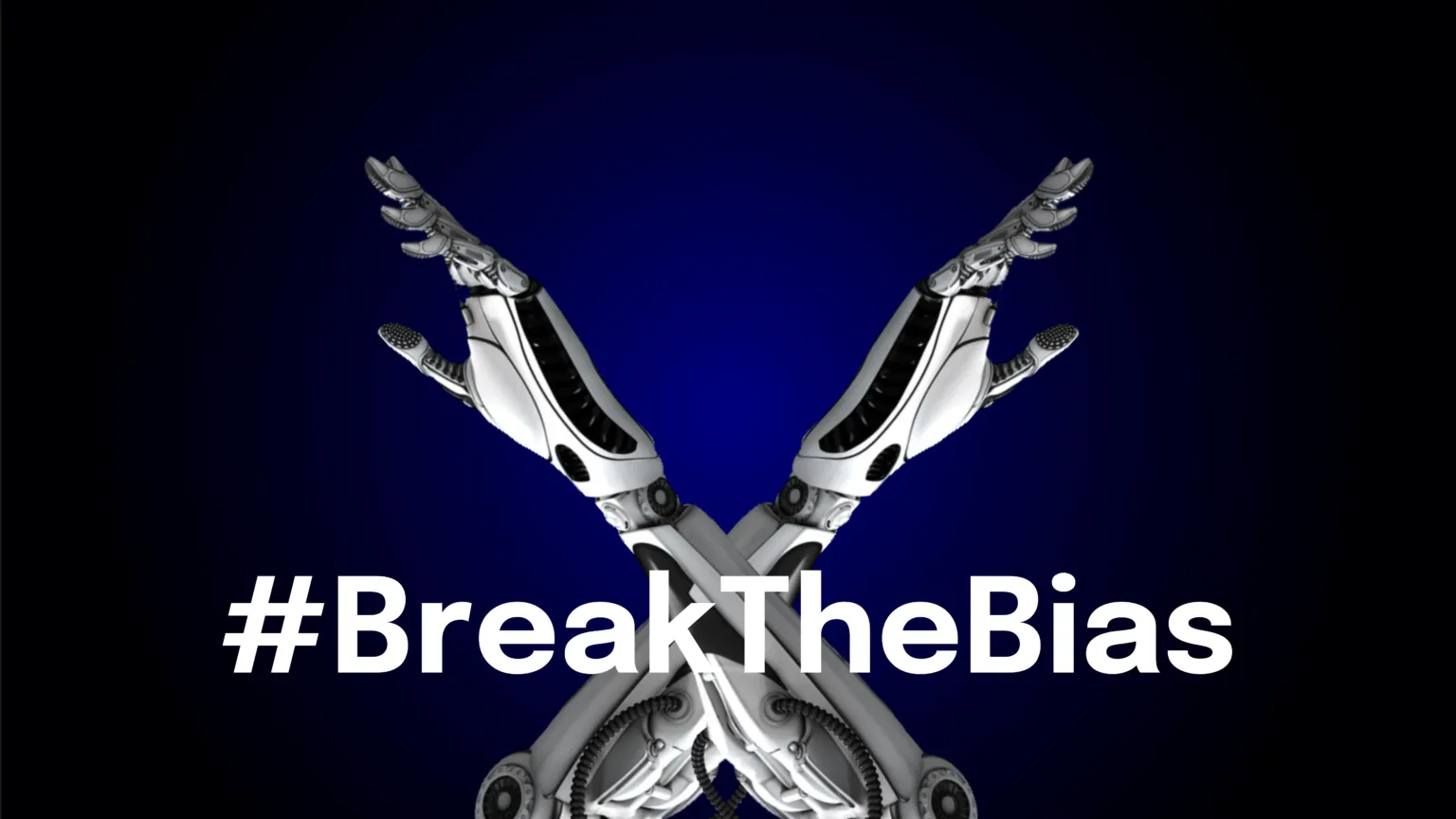
We all have such unconscious biases, and since we are all different people, these biases vary from person to person. However, since the current community of AI developers comprises over 80% white cis-men, the values, ideas, and biases creeping into AI systems are very homogeneous and thus literally narrow-minded. Starting with the definition of AI, the founding fathers of AI back in 1956 were all white male engineers, a very homogeneous group of people, which led to a narrow idea of what intelligence is, namely the ability to win games such as chess. However, from psychology, we know that there are a lot of different kinds of intelligence, such as emotional or social intelligence. Still, today, if a model is developed and reviewed by a very homogenous group of people, without special attention and processes, they will not be able to identify discrimination who are different from themselves due to unconscious biases. Indeed, this homogenous community tends to be the group of people who barely suffer from bias in AI.
Just imagine if all the children in the world were raised and educated by 30-year-old white cis-men. That is what our AI looks like today. It is designed, developed, and evaluated by a very homogenous group, thus, passing on a one-sided perspective on values, norms, and ideas. Developers are at the core of this. They are teaching AI what is right or wrong, what is good or bad.
Break the Bias in Society
So, a crucial step towards fair and unbiased AI is a diverse and inclusive AI development community. Meanwhile, there are some technical solutions to the mentioned data and model bias problems (e.g., data diversification or causal modeling). Still, all these solutions are useless if the developers fail to think about bias problems in the first place. Diverse people can better check each other’s blindspots, each other’s biases. Many studies show that diversity in data science teams is critical in reducing bias in AI.
Furthermore, we must educate our society on AI, its risks, and its chances. We need to rethink and restructure the education of AI developers, as they need as much ethical knowledge as technical knowledge to develop fair and unbiased AI systems. We need to educate the broad population that we all can also become part of this massive transformation through AI to contribute our ideas and values to the design and development of these systems.
In the end, if we want to break the bias in AI, we need to break the bias in our society. Diversity is the solution to fair and unbiased AI, not only in AI developing teams but across our whole society. AI is made by humans, by us, by our society. Our society with its structures brings bias in AI: through the data we produce, the goals we expect the machines to achieve and the community developing these systems. At its core, bias in AI is not a technical problem – it is a social one.
Positive Reinforcement of AI
Finally, we need to ask ourselves: do we want AI reflecting society as it is today or a more equal society of tomorrow? Suppose we are using machine learning models to replicate the world as it is today. In that case, we are not going to make any social progress. If we fail to take action, we might lose some social progress, such as more gender equality, as AI amplifies and reinforces bias back into our lives. AI is supposed to be forward-looking. But at the same time, it is based on data, and data reflects our history and present. So, as much as we need to break the bias in society to break the bias in AI systems, we need unbiased AI systems for social progress in our world.
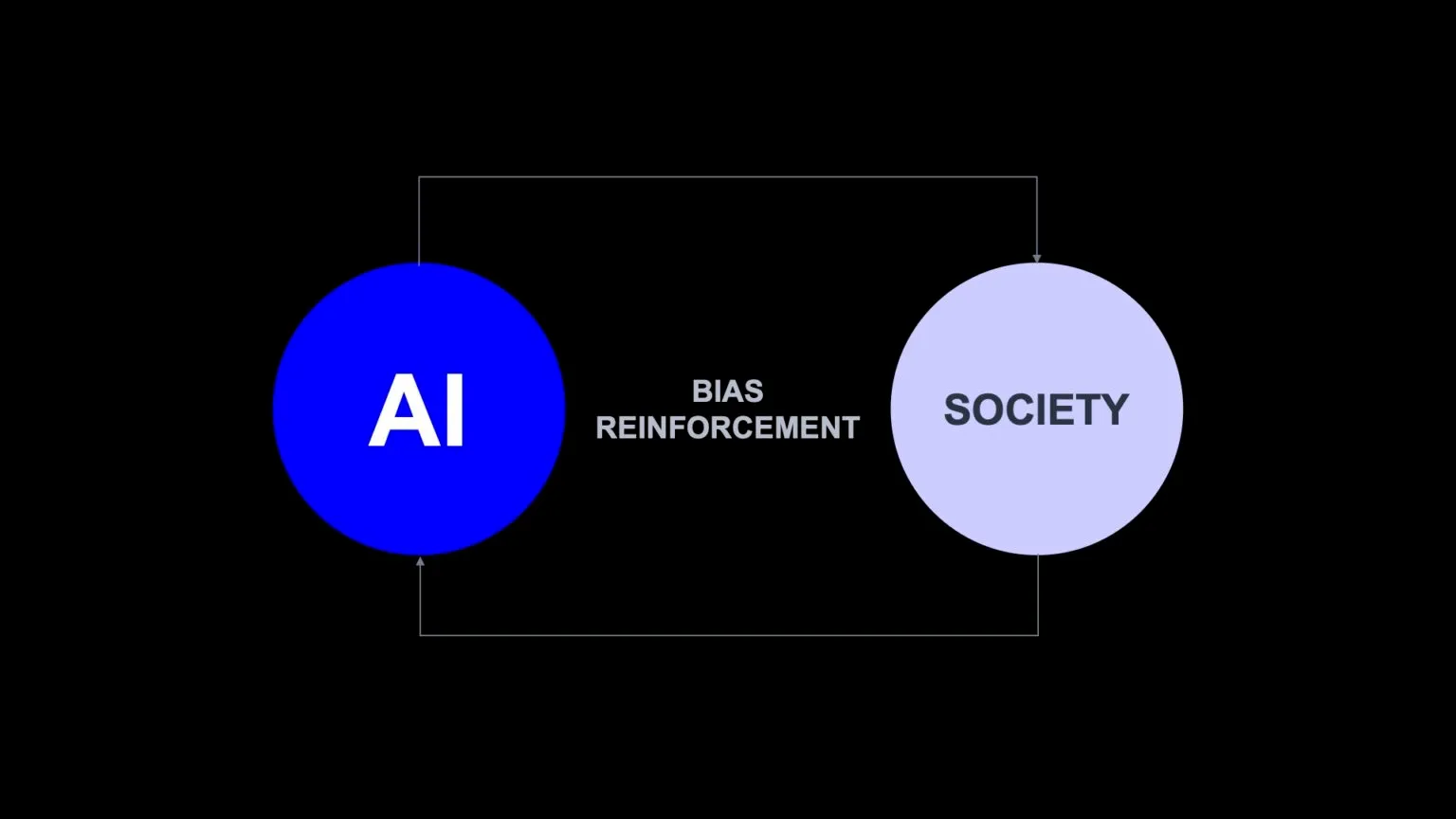
Having said all that, I am hopeful and optimistic. Through this amplification effect, AI has raised awareness of old fairness and discrimination issues in our society on a much broader scale. Bias in AI shows us some of the most pressing societal challenges. Ethical and philosophical questions become ever more important. And because AI has this reinforcement effect on society, we can also use it for the positive. We can use this technology for good. If we all work together, it is our chance to remake the world into a much more diverse, inclusive, and equal place.