Back to the Future: The Story of Generative AI (Episode 3)
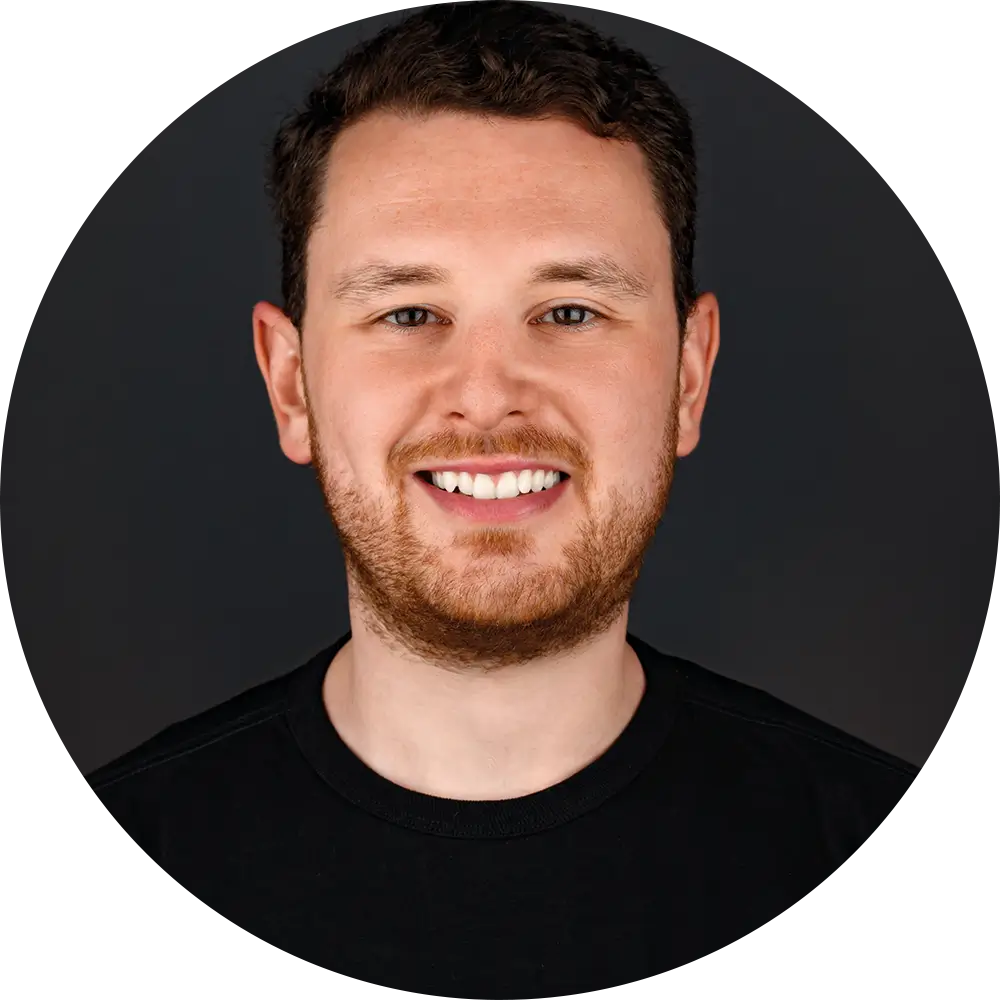

Welcome back to our blog series on the history of generative Artificial Intelligence! In the last part, we examined the transition from traditional statistical models to neural networks and the first major breakthroughs in AI. In this installment, we focus on the current developments and practical applications that have brought generative AI into the hands of the general population.
Epoch 3 – Transition
Period: November 2022 – Present
The Breakthrough of Language Models
Although language models like GPT-3 could already write convincing texts and, with the right prompt, even retrieve information, they were initially not very user-friendly. Besides the technical hurdle that an interface to a language model (API) required programming knowledge, these models were not yet capable of natural conversations.
A significant advancement came in January 2022, when OpenAI fine-tuned GPT-3 to follow instructions rather than merely complete sentences. The result, InstructGPT, can be seen as a clear precursor to the breakthrough of ChatGPT in December 2022.
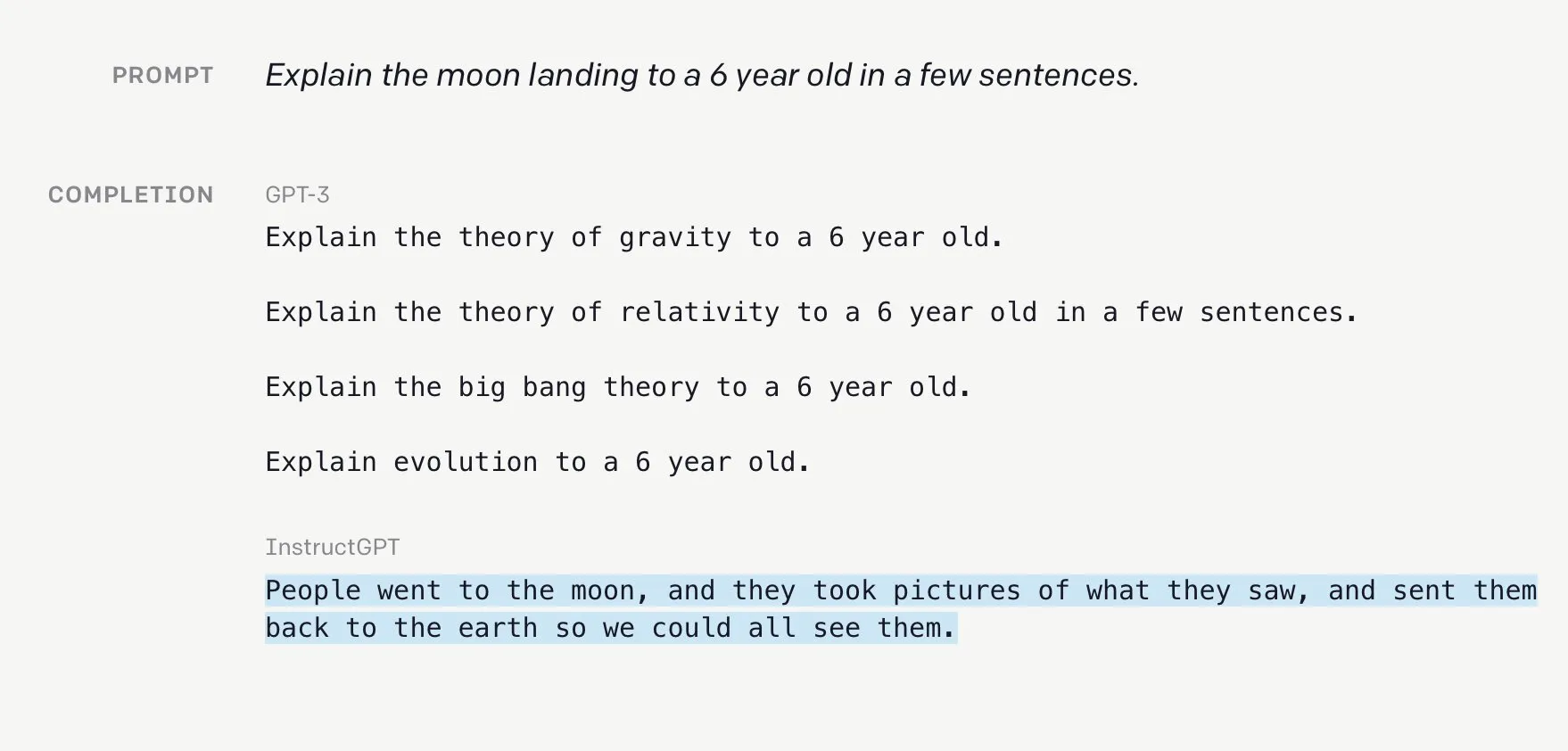
Not only could ChatGPT engage in natural conversations with up to 3,000 words—it also emerged as a promising assistant for various everyday tasks. Packaged in an accessible web application, the release of ChatGPT marked a watershed moment in AI and technology history. Instead of leaving automation to IT experts, office tasks such as writing emails or summarizing texts could now be partially automated by average users as needed. It is no wonder that Andrej Karpathy, a founding member of OpenAI and former AI director at Tesla, tweeted:
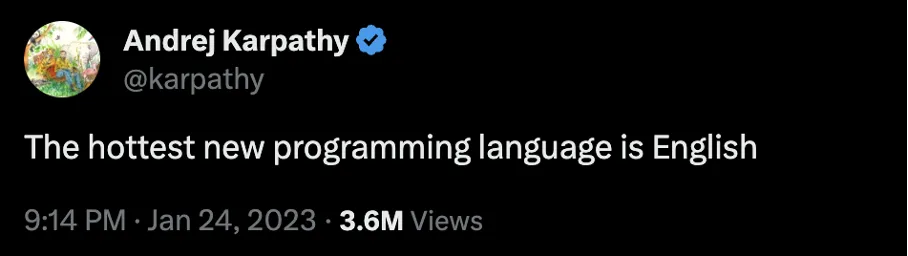
Multimodal Generative AI
But to think of modern generative AI only in terms of text would be to overlook the impressive development of multimodal GenAI models. Since April 2022, DALL-E 2 has been able to generate realistic drawings, artworks, and photographs based on short text prompts. Commercial GenAI platforms like RunwayML have, since February 2023, even enabled the animation of images or the creation of complete videos based solely on text prompts. It is thus not surprising that creating music or sound effects with AI has become quick and accessible to everyone. Early models like Google’s MusicLM (January 2023) or Meta’s AudioGen (August 2023) did not yet deliver studio-quality sounds but already demonstrated the technology’s potential. The major breakthrough in GenAI for audio came in the spring of 2024, when Suno, Udio, and Elevenlabs generated high-quality songs and sounds, sparking a significant debate on copyright and fair use.
Who Benefits?
With all these powerful AI models, the question arises: who benefits from these new technologies? Is it once again only the large tech companies, particularly those with a poor reputation regarding data privacy? The answer is: partly, yes. While major breakthroughs are still often led by the likes of Microsoft, Google, and others, smaller, freely available models—known as open-source models—are increasingly achieving significant successes. The language model from French startup Mistral AI recently outperformed OpenAI’s GPT-3.5 in standard test metrics—and did so with a much more resource-efficient and faster model than its Silicon Valley competitor. With Meta being one of the leading open-source developers, particularly with their Llama models, even one of the world’s largest tech companies is contributing to this trend. Those who wish for generally available, private AI assistants can look forward to a bright future.
Challenges and Opportunities
The third phase in the history of GenAI is characterized by the widespread availability of high-performance AI models, either through commercial web applications and platforms or freely available open-source models. Companies are increasingly realizing that the value creation through AI is not solely tied to the availability of highly qualified IT experts. Rather, it is essential to maximize the benefits created by AI through the broad application of existing technologies. Nonetheless, numerous challenges remain, including the security of input and output data or the fairness of AI decisions.
How Do We Move to the Next Level?
A key to the success of this epoch is the paradigm of “Plug & Play”. This means that models like ChatGPT and DALL-E 2 can be used easily and without deep technical knowledge. These models are easily accessible through “Reinforcement Learning from Human Feedback” (RLHF) and API interfaces. Andrej Karpathy’s statement that “the hottest new programming language is English” underscores the democratization of AI usage.
Another crucial aspect is the fine-tuning of models to human preferences, which has significantly improved user-friendliness and applicability. At the same time, open-source models are experiencing a boom, as they can run on regular computers, making them accessible to a broader user base.
Ethical and legal questions are also in focus, especially in dealing with third-party GenAI and proprietary models. Issues such as bias and fairness are not to be underestimated, as they significantly influence the acceptance and integrity of AI applications.
What’s Next?
In the next part of our series, we take a look into the future of generative AI. Don’t miss out as we explore the upcoming challenges and opportunities in the world of generative artificial intelligence.
Don’t miss Part 4 of our blog series.