3 specific use cases of how ChatGPT will revolutionize communication in companies

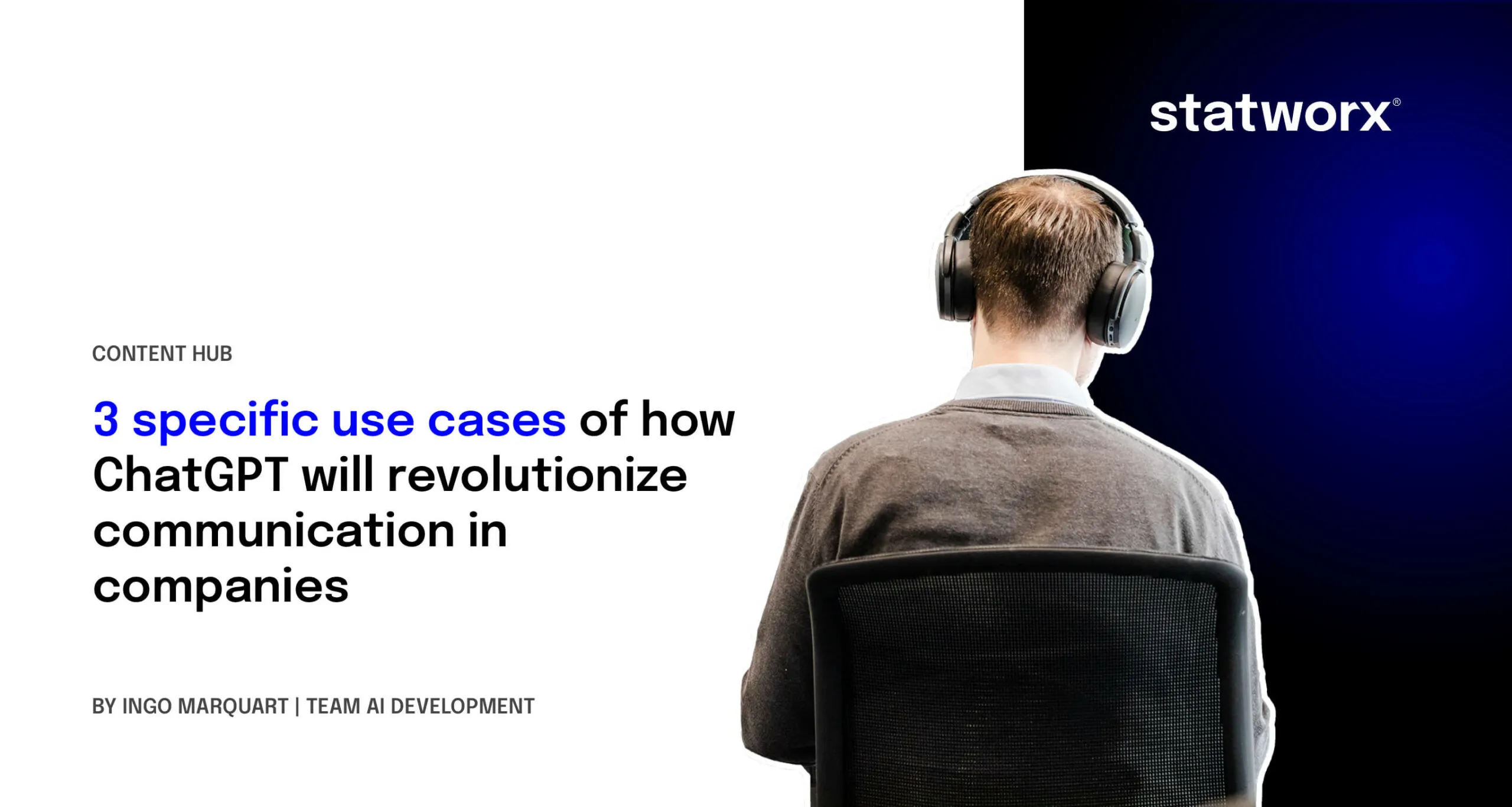
It’s no secret that the latest language models like ChatGPT have far exceeded our wildest expectations. It’s impressive and even somewhat eerie to think that a language model possesses both a broad knowledge base and the ability to convincingly answer (almost) any question. Just a few hours after the release of this model, speculation began about which fields of activity could be enriched or even replaced by these models, which use cases can be implemented, and which of the many new start-up ideas arising from ChatGPT will prevail.
There is no doubt that the continuous development of artificial intelligence is gaining momentum. While ChatGPT is based on a third-generation model, a “GPT-4” is already on the horizon, and competing products are also waiting for their big moment.
As decision-makers in a company, it is now important to understand how these advancements can actually be used to add value. In this blog post, we will focus on the background instead of the hype, provide examples of specific use cases in corporate communication, and particularly explain how the implementation of these AI systems can be successful.
What is ChatGPT?
If you ask ChatGPT this question, you will receive the following answer:
“ChatGPT is a large language model trained by OpenAI to understand and generate natural language. It uses deep learning and artificial intelligence technology to conduct human-like conversations with users.”
ChatGPT is the latest representative from a class of AI systems that process human language (i.e. texts). This is called “Natural Language Processing”, or NLP for short. It is the product of a whole chain of innovations that began in 2017 with a new AI architecture. In the following years, the first AI models were developed based on this architecture that reached human-level language understanding. In the last two years, the models learned to write and even have whole conversations with the user using ChatGPT. Compared to other models, ChatGPT stands out for generating credible and appropriate responses to user requests.
In addition to ChatGPT, there are now many other language models in different forms: open source, proprietary, with dialog options or other capabilities. It quickly became apparent that these capabilities continued to grow with larger models and more (especially high-quality) data. Unlike perhaps originally expected, there seems to be no upper limit. On the contrary, the larger the models, the more capabilities they gain!
These linguistic abilities and the versatility of ChatGPT are amazing, but the use of such large models is not exactly resource-friendly. Large models like ChatGPT are operated by external providers who charge for each request to the model. In addition, every request to larger models not only generates more costs but also consumes more electricity and thus affects the environment.
For example, most chat requests from customers do not require comprehensive knowledge of world history or the ability to give amusing answers to every question. Instead, existing chatbot services tailored to business data can deliver concise and accurate answers at a fraction of the cost.
Modern Language Models in Business Applications
Despite the potential environmental and financial costs associated with large language models like ChatGPT, many decision-makers still want to invest in them. The reason lies in their integration into organizational processes. Large generative models like ChatGPT enable us to use AI in every phase of business interaction: incoming customer communication, communication planning and organization, outgoing customer communication and interaction execution, and finally, process analysis and improvement.
In the following, we will explain in detail how AI can optimize and streamline these communication processes. It quickly becomes clear that it is not just a matter of applying a single advanced AI model. Instead, it becomes apparent that only a combination of multiple models can effectively address the challenges and deliver the desired economic benefits in all phases of interaction.
For example, AI systems are becoming increasingly relevant in communication with suppliers or other stakeholders. However, to illustrate the revolutionary impact of new AI models more concretely, let us consider the type of interaction that is vital for every company: communication with the customer.
Use Case 1: Incoming Customer Communication with AI.
Challenge
Customer inquiries are received through various channels (emails, contact forms on the website, apps, etc.) and initiate internal processes and workflows within the CRM system. Unfortunately, the process is often inefficient and leads to delays and increased costs due to inquiries being misdirected or landing in a single central inbox. Existing CRM systems are often not fully integrated into organizational workflows and require additional internal processes based on organic routines or organizational knowledge of a small number of employees. This reduces efficiency and leads to poor customer satisfaction and high costs.
Solution
Customer communication can be a challenge for businesses, but AI systems can help automate and improve it. With the help of AI, the planning, initiation, and routing of customer interactions can be made more effective. The system can automatically analyze content and information and decide how best to handle the interaction based on appropriate escalation levels. Modern CRM systems are already able to process standard inquiries using low-cost chatbots or response templates. But if the AI recognizes that a more complex inquiry is present, it can activate an AI agent like ChatGPT or a customer service representative to take over the communication.
With today’s advances in the NLP field, an AI system can do much more. Relevant information can be extracted from customer inquiries and forwarded to the appropriate people in the company. For example, a key account manager can be the recipient of the customer message while a technical team is informed with the necessary details. In this way, more complex scenarios, the organization of support, the distribution of workloads, and the notification of teams about coordination needs can be handled. These processes are not manually defined but learned by the AI system.
More details such as technical architecture, vendors, pros and cons of models, requirements and timelines can be found in the following download:
The implementation of an integrated system can increase the efficiency of a company, reduce delays and errors, and ultimately lead to higher revenue and profit.
Use Case 2: Outgoing Customer Communication with AI.
Challenge
Customers expect their inquiries to be promptly, transparently, and precisely answered. A delayed or incorrect response, a lack of information, or uncoordinated communication between different departments are breaches of trust that can have a negative long-term effect on customer relationships.
Unfortunately, negative experiences are commonplace in many companies. This is often because existing solutions’ chatbots use standard answers and templates and are only rarely able to fully and conclusively answer customer inquiries. In contrast, advanced AI agents like ChatGPT have higher communication capabilities that enable smooth customer communication.
When the request does reach the right customer service employees, new challenges arise. Missing information regularly leads to sequential requests between departments, resulting in delays. Once processes, intentionally or unintentionally, run in parallel, there is a risk of incoherent communication with the customer. Ultimately, both internally and externally, transparency is lacking.
Solution
AI systems can support companies in all areas. Advanced models like ChatGPT have the necessary linguistic abilities to fully process many customer inquiries. They can communicate with customers and at the same time ask internal requests. This makes customers no longer feel brushed off by a chatbot. The technical innovations of the past year allow AI agents to answer requests not only faster but also more accurately. This relieves the customer service and internal process participants and ultimately leads to higher customer satisfaction.
AI models can also support human employees in communication. As mentioned at the beginning, there is often simply a lack of accurate and precise information available in the shortest possible time. Companies are striving to break down information silos to make access to relevant information easier. However, this can lead to longer processing times in customer service, as the necessary information must first be collected. An essential problem is that information can be presented in various forms, such as text, tabular data, in databases, or even in the form of structures like previous dialogue chains.
Modern AI systems can handle unstructured and multimodal information sources. Retrieval systems connect customer requests to various information sources. The additional use of generative models like GPT-3 then allows the found information to be efficiently synthesized into understandable text. Individual “Wikipedia articles” can be generated for each customer request. Alternatively, the customer service employee can ask a chatbot for the necessary information, which is immediately and understandably provided.
It is evident that an integrated AI system not only relieves customer service but also other technical departments. This type of system has the potential to increase efficiency throughout the company.
Use Case 3: Analysis of communication with AI
Challenge
Robust and efficient processes do not arise on their own, but through continuous feedback and constant improvements. The use of AI systems does not change this principle. An organization needs a process of continuous improvement to ensure efficient internal communication, effectively manage delays in customer service, and conduct result-oriented sales conversations.
However, in outward dialogue, companies face a problem: language is a black box. Words have unparalleled information density precisely because their use is deeply rooted in context and culture. This means that companies evade classical statistical-causal analysis because communication nuances are difficult to quantify.
Existing solutions therefore use proxy variables to measure success and conduct experiments. While overarching KPIs such as satisfaction rankings can be extracted, they must be obtained from the customer and often have little meaning. At the same time, it is often unclear what can be specifically changed in customer communication to modify these KPIs. It is difficult to analyze interactions in detail, identify dimensions and levers, and ultimately optimize them. The vast majority of what customers want to reveal about themselves immediately exists in text and language and evades analysis. This problem arises both with the use of AI assistance systems and with customer service representatives.
Solution
While modern language models have received much attention due to their generative capabilities, their analytical capabilities have also made enormous strides. The ability of AI models to respond to customer inquiries demonstrates an advanced understanding of language, which is essential for improving integrated AI systems. Another application is the analysis of conversations, including the analysis of customers and their own employees or AI assistants.
By using artificial intelligence, customers can be more precisely segmented by analyzing their communication in detail. Significant themes are captured and customer opinions are evaluated. Semantic networks can be used to identify which associations different customer groups have with products. In addition, generative models are used to identify desires, ideas, or opinions from a wealth of customer voices. Imagine being able to personally go through all customer communication in detail instead of having to rely on synthetic KPIs – that’s exactly what AI models make possible.
Of course, AI systems also offer the ability to analyze and optimize their own processes. AI-supported dialogue analysis is a promising area of application that is currently being intensively researched. This technology enables, for example, the examination of sales conversations with regard to successful closures. Breaking points in the conversation, changes in mood and topics are analyzed to identify the optimal course of a conversation. This type of feedback is extremely valuable not only for AI assistants but also for employees because it can even be played during a conversation.
In summary, the use of AI systems improves the breadth, depth, and speed of feedback processes. This allows the organization to be agile in responding to trends, desires, and customer opinions and to further optimize internal processes.
More details such as technical architecture, vendors, pros and cons of models, requirements and timelines can be found in the following download:
Obstacles to consider when implementing AI systems
The application of AI systems has the potential to fundamentally revolutionize communication with customers. Similar potential can also be demonstrated in other areas, such as procurement and knowledge management, which are discussed in more detail in the accompanying materials.
However, it is clear that even the most advanced AI models are not yet ready for deployment in isolation. To move from experimentation to effective implementation, it takes experience, good judgment, and a well-coordinated system of AI models.
The integration of language models is even more important than the models themselves. Since language models act as the interface between computers and humans, they must meet specific requirements. In particular, systems that intervene in work processes must learn from the established structures of the company. As interface technology, aspects such as fairness, impartiality, and fact-checking must be integrated into the system. In addition, the entire system needs a direct intervention capability for employees to identify errors and realign the AI models if necessary. This “active learning” is not yet standard, but it can make the difference between theoretical and practical efficiency.
The use of multiple models that run both on-site and directly from external providers presents new demands on the infrastructure. It is also important to consider that essential information transfer is not possible without careful treatment of personal data, especially when critical company information must be included. As described earlier, there are now many language models with different capabilities. Therefore, the solution’s architecture and models must be selected and combined according to the requirements.
Finally, there is the question of whether to rely on solution providers or develop your own (partial) models. Currently, there is no standard solution that meets all requirements, despite some marketing claims. Depending on the application, there are providers of cost-effective partial solutions. Making a decision requires knowledge of these providers, their solutions, and their limitations.
Conclusion
In summary, it can be stated that the use of AI systems in customer communication can improve and automate processes. A central objective for companies should be the optimization and streamlining of their communication processes. AI systems can support this by making the planning, initiation, and forwarding of customer interactions more effective and by either activating a chatbot like ChatGPT or a customer service representative for more complex inquiries. By combining different models in a targeted manner, meaningful problem-solving can be achieved in all phases of interaction, generating the desired economic benefits.
More details such as technical architecture, vendors, pros and cons of models, requirements and timelines can be found in the following download: