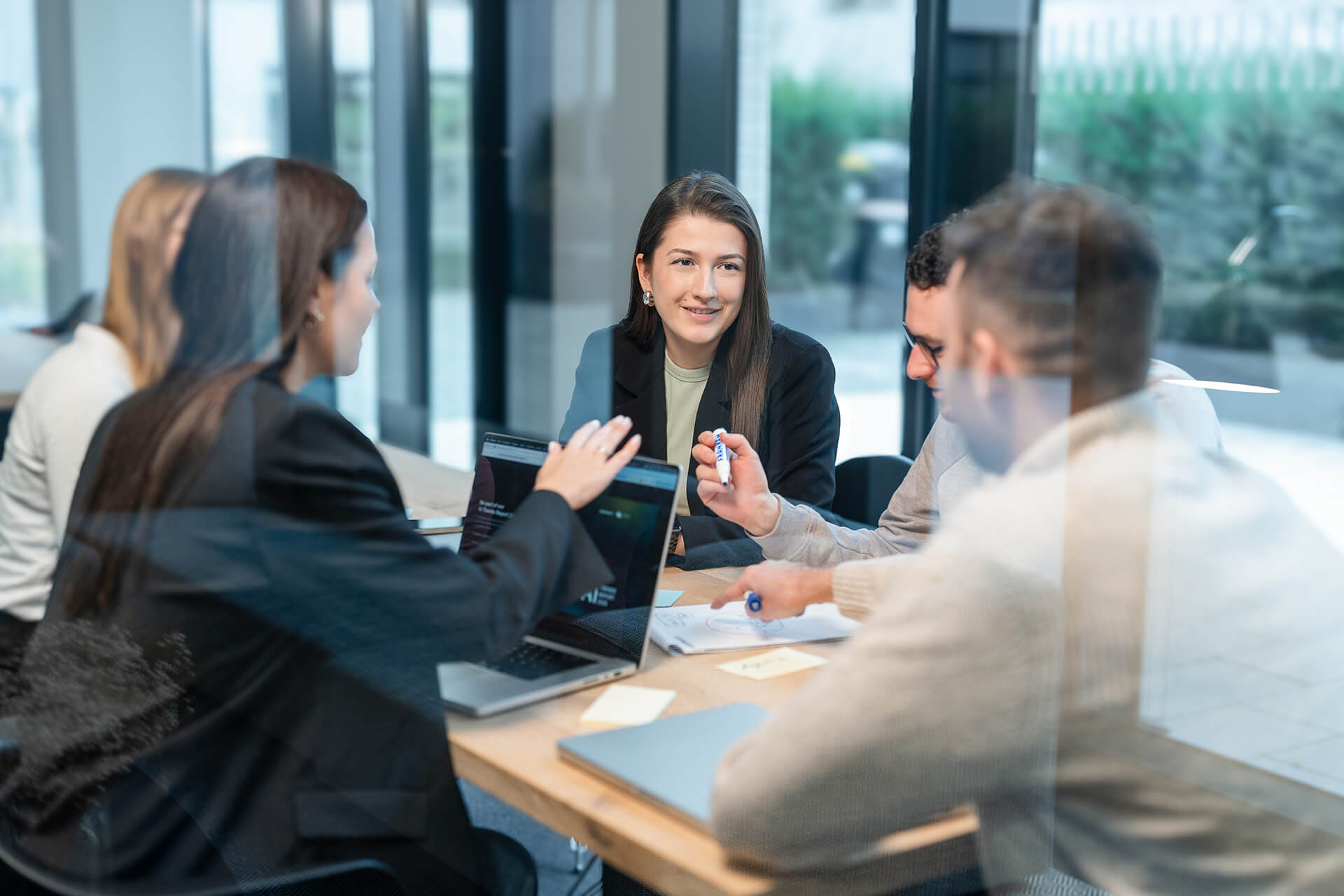

statworx ist eines der führenden Beratungs- und Entwicklungsunternehmen für Daten und KI in der DACH-Region. Wir sind mehr als ein Dienstleister – wir sind Ihr Partner für die gesamte KI-Transformation. Wir beraten, wir entwickeln, wir bilden weiter – seit mehr als 10 Jahren, in über 1.000 Daten- & KI-Projekten und für über 100 Kunden aus fast allen Industrien. Unsere Expert:innen verstehen, welche Tech-Trends Ihr Unternehmen wirklich besser machen.