Upselling of Insurance Policies
As part of an annual upselling campaign, we collaborated with our client to develop a model for predicting product-affine customers. The model was subsequently used to optimize customer selection.
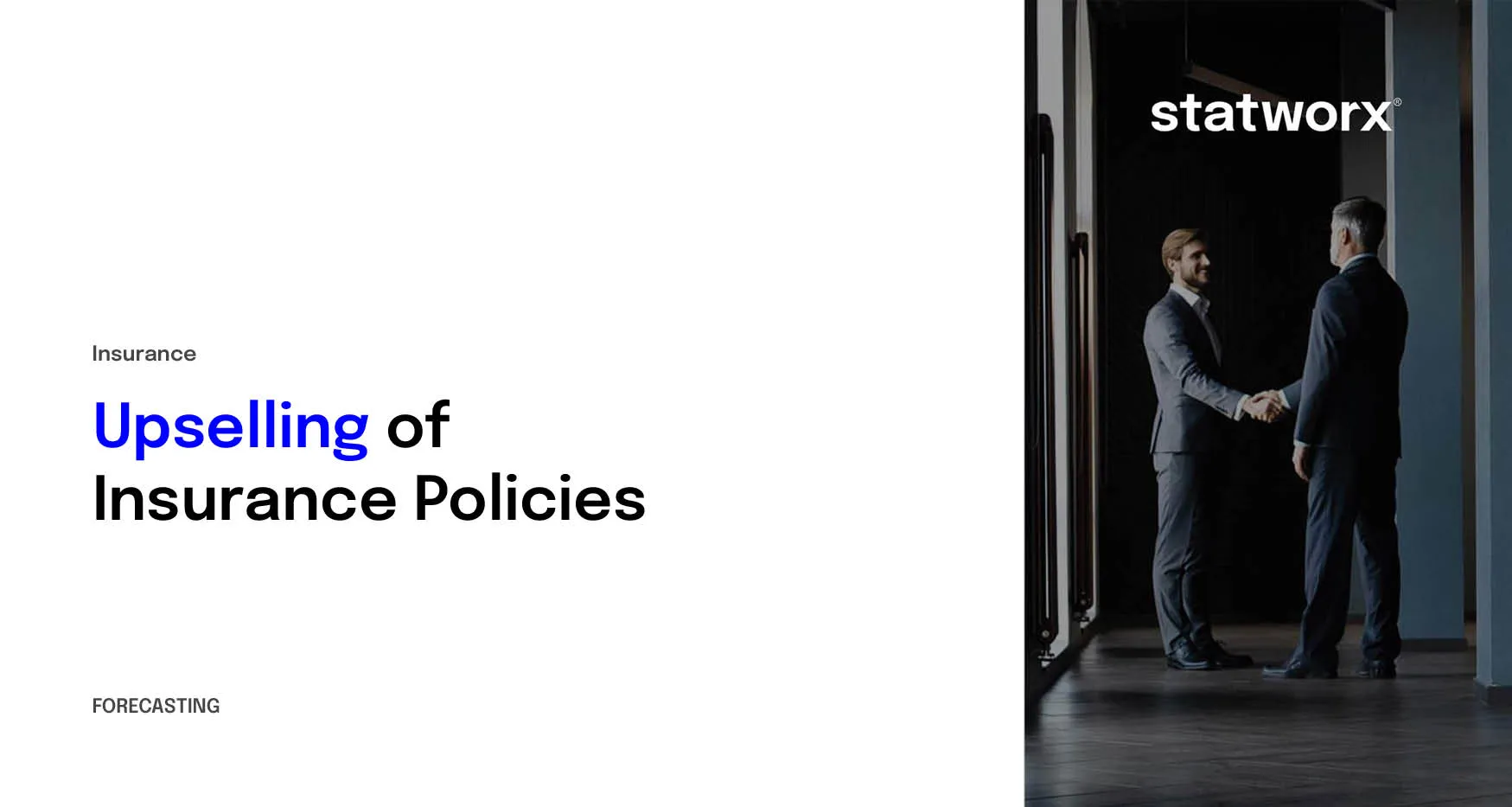
Challenge
In the realm of long-term insurance products such as life insurance, insurers face the problem of having limited contact with policyholders between the initiation of the policy and any potential claims. Due to the lack of touchpoints, there are fewer opportunities for successful upselling compared to other types of insurance. Our client, an international insurance company, annually activates existing upselling potentials by sending offers to increase the insurance sum accordingly. The distribution of these high-quality letters is costly and has so far yielded only average responses.
Approach
After exploring and preparing historical customer information from various IT systems, we worked with the client to develop a machine learning algorithm. Based on the response behavior of all customers in previous years, this algorithm can distinguish potential upsellers from other customers with high accuracy. The model is based on "gradient boosting," a state-of-the-art method in machine learning. The model was implemented into the client's IT infrastructure and can now be run automatically on-demand or at regular intervals.
Result
Through the first application of the model in the field and the generated forecasts at the customer level, over 90% of the additional payments were generated with a fraction of the previous year's mailing budget, representing a multiplication of the response rate and thus effectiveness.