Prediction of Online Conversions
In this project, we developed a machine learning model to predict conversion potential based on individual customer journey data on the client's side.
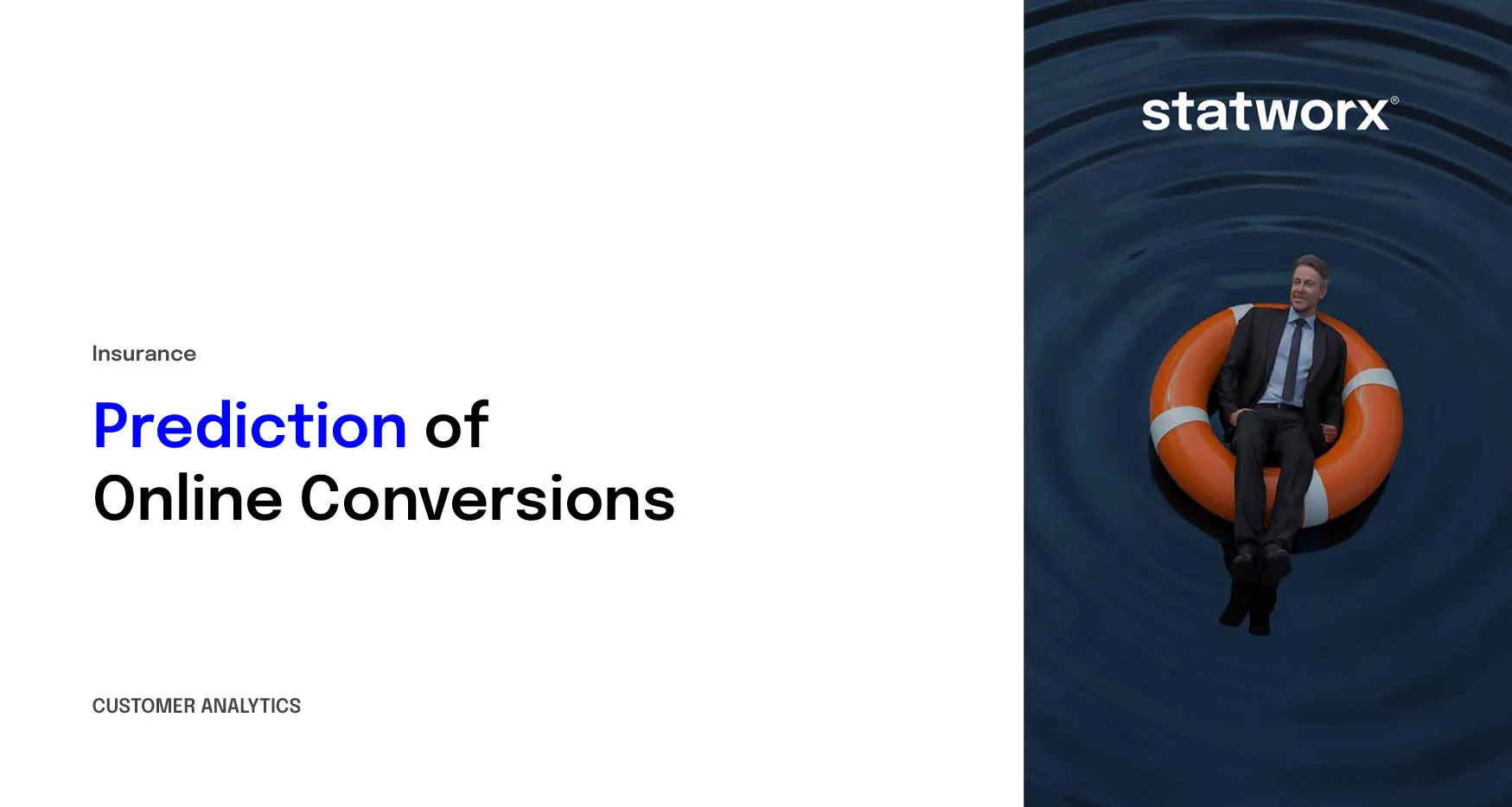
Challenge
Modeling customer journey data is a central issue in web analytics. For our client, an online insurance company that had previously only worked with simple attribution models, questions about customer behavior along the journey and the modeling of conversions posed new challenges to be addressed within the project.
Approach
Based on tens of thousands of individual customer journeys generated through the client's own web tracking system, we developed a machine learning model that can predict conversions on the client's website with high accuracy using historical online contact points across various channels and websites. The model utilizes all available digital touchpoints to make the most accurate prediction possible. Notably, the entire customer journey, not just the last contact point before conversion, is incorporated into the prediction. The model self-trains using "gradient boosting," a state-of-the-art method in machine learning.
Result
By modeling the entire customer journey data available to the client, it was demonstrated for the first time that conversion prediction is possible and depends on many factors beyond the last touchpoint. Scoring the cookies can be used to optimize online bidding strategies or to present targeted offers in real-time on the website.