Data Product Operationalization
In this project, we developed various deployment scenarios for an existing forecasting model of our client.
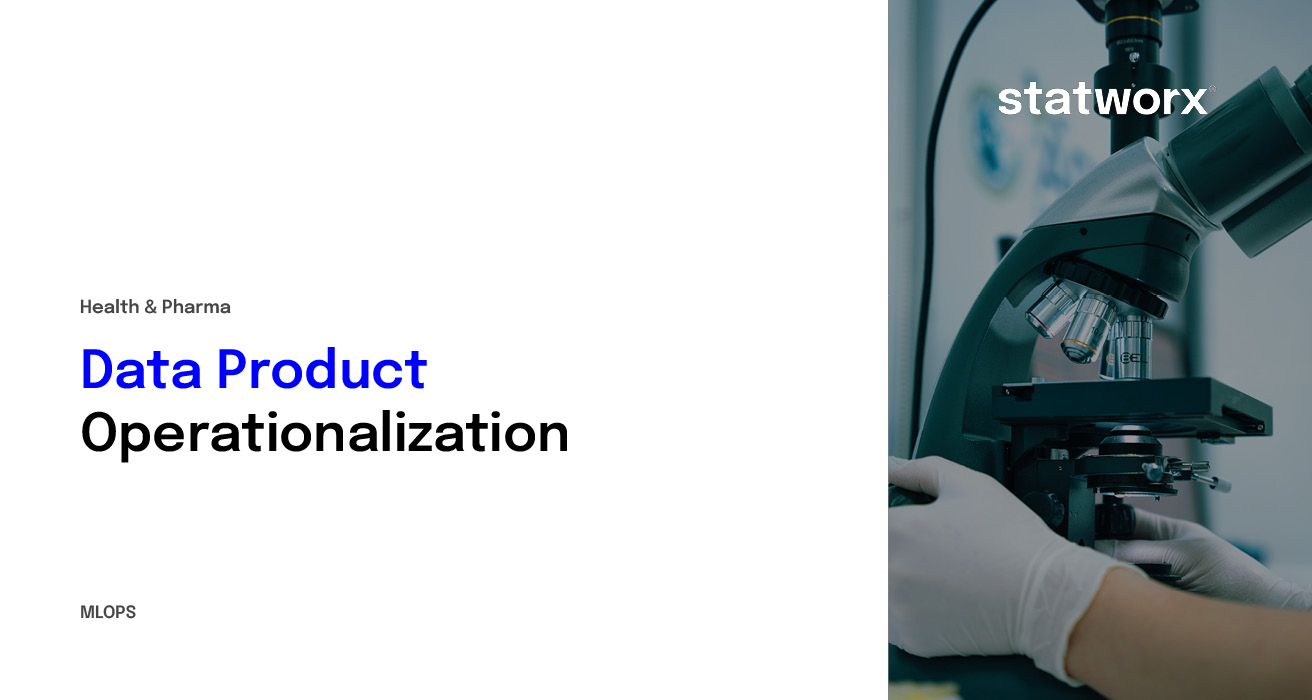
Challenge
Transitioning machine learning prototypes or MVPs into a production environment is a central challenge in the end-to-end data science process. Our client, an international pharmaceutical and healthcare corporation, faced the challenge of migrating one of its data products from the forecasting domain from a prototype environment to the new cloud platform. The focus was particularly on exploring the options, as well as the advantages and disadvantages, regarding potential target architectures.
Approach
In several exploration and deep dive workshops, our machine learning and data engineers analyzed the source code and the current workflow of the data product. Subsequently, we collaborated with the client's data platform and ML engineers to develop several deployment blueprints. Each blueprint highlighted a possible architecture target, varying the proportions of cloud services and open-source components used.
Result
Our client could choose from three different production scenarios. A blueprint composed solely of cloud services was selected. In a follow-up project, the blueprint was implemented on the client's cloud platform, thus operationalizing the machine learning model.