Prediction of Customer Churn
In this project, we developed a model based on historical customer data to predict customer churn and identify its influencing factors.
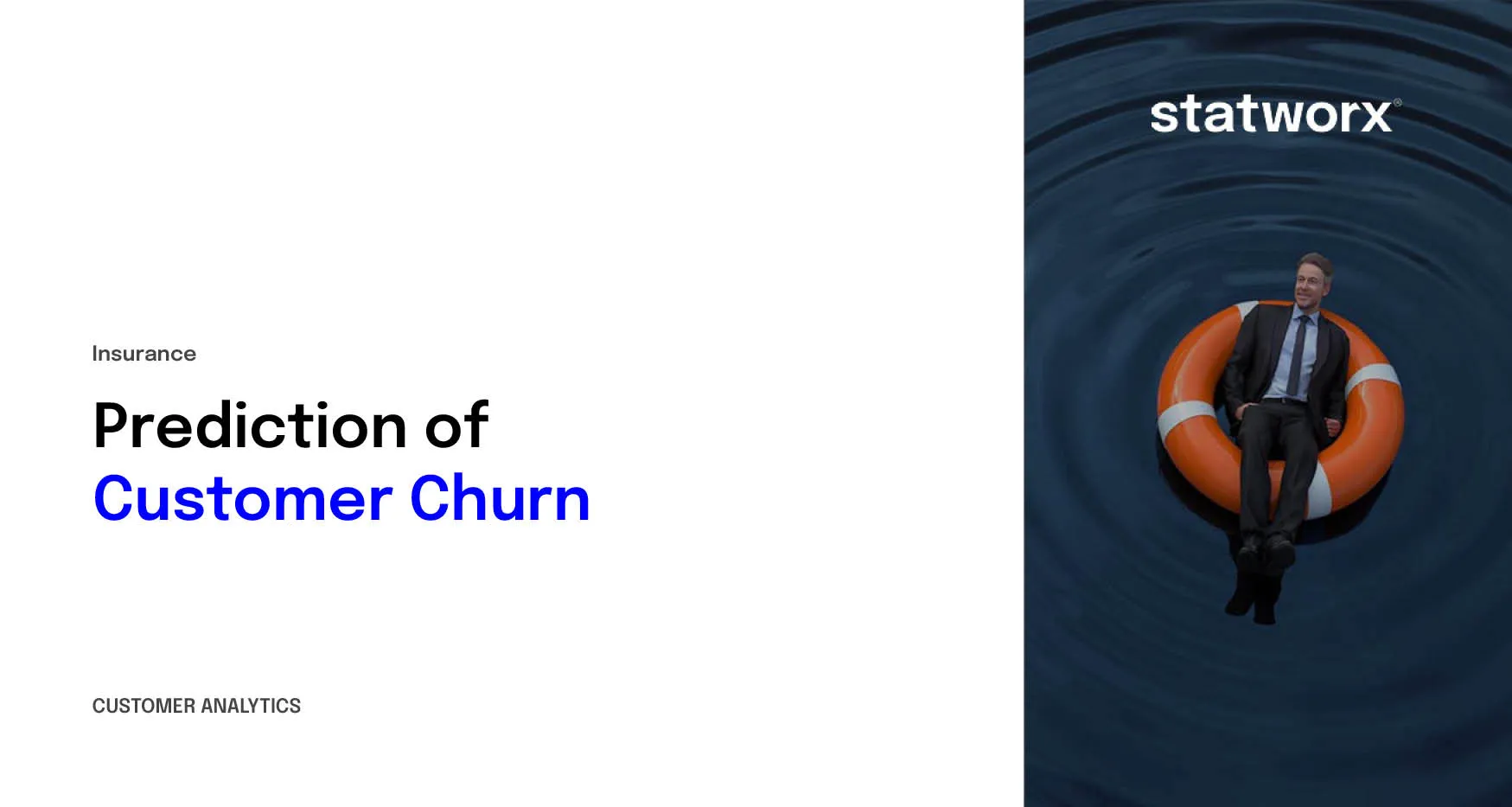
Challenge
Customer churn presents various disadvantages for companies. A direct impact is the loss of payments. In the long term, each churn also means lost opportunities for upselling or generating new customers through referrals. Our client, an international insurance company, wanted to better understand which customers were churning and why. Additionally, they sought to create a model for predicting churn.
Approach
To model this question, we utilized the client's existing data warehouse structures, which contained extensive information on various customer characteristics. Since customer churn is a time-point decision, merging the different data sources created a consolidated panel data structure that captured changes in customer characteristics over time (e.g., changes in marital status, etc.). Based on this data, we developed a machine learning model to identify customers at risk of churning over time, which was then implemented into the client's IT infrastructure.
Result
The model automatically generates churn metrics for all active customers on a weekly basis at the database level. These metrics are used in various parts of the company to optimize customer engagement concerning churn and to initiate appropriate countermeasures.